Lithium ion battery thermal process space-time modeling method based on dual-scale manifold learning
A lithium-ion battery and manifold learning technology, which is applied in the field of lithium-ion battery thermal process research, can solve problems such as the imperfect structure of the manifold structure graph and the large amount of calculation
- Summary
- Abstract
- Description
- Claims
- Application Information
AI Technical Summary
Problems solved by technology
Method used
Image
Examples
Embodiment 1
[0089] Such as figure 1 As shown, the spatio-temporal modeling method of lithium-ion battery thermal process based on dual-scale manifold learning includes the following steps:
[0090] S1: According to the manifold learning method, construct a set of nonlinear spatial basis functions for time / space separation;
[0091] S2: Use the Galerkin method to truncate the nonlinear space basis function to obtain a physics-based time model;
[0092] S3: Use the extreme learning machine to evaluate and learn the unknown model structure and parameters existing in the time model;
[0093] S4: Based on the nonlinear spatial basis function and temporal model, the spatial-temporal model of LIBs is reconstructed using the spatio-temporal synthesis method.
[0094] Wherein, the concrete process of described step S1 comprises:
[0095] S11: Construct neighbor graphs and supplementary graphs to represent the manifold structure in the original space;
[0096] S12: Calculate the local linear we...
Embodiment 2
[0151] More specifically, the implementation of the scheme is carried out by taking 60Ah LiFePO4 / graphite rechargeable lithium-ion batteries (LIBs) as an example.
[0152] In the specific implementation process, the battery is regarded as a two-dimensional distribution heat process, and the temperature difference along the thickness direction is not considered. 20 thermocouple sensors are located on the surface of the battery for temperature data acquisition. Such as figure 2 As shown, the sensors marked with "cross" are used for model estimation, while the sensors marked with "circle" are used for model validation. In this experiment, the battery is subjected to cyclic charge and discharge experiments by the battery thermal system (BTS) integrated battery tester, incubator, battery management system (BMS) and host computer, such as image 3 shown. The input current and corresponding measured voltage can be measured with an integrated battery meter.
[0153] In the specif...
Embodiment 3
[0156] More specifically, in order to verify the performance of the model, the present invention uses three commonly used spatial BFs optimal learning methods for comparison: local manifold learning (LLE), global manifold learning (ISOMAP) and Karhunen–Loève (KL) method . The first two methods only consider a single nonlinear spatial information in the process of model dimensionality reduction, and the third method is a linear model dimensionality reduction technique. In order to facilitate comparison with other methods, the present invention introduces five error indicators. The role of these indicators can be categorized as follows:
[0157] (1) Spatio-temporal prediction error (SPE): evaluates the deviation between the model output value and the measured output value.
[0158]
[0159] (2) Mean Square Error (RMES): Similar to SPE, this indicator is also used to evaluate prediction bias. However, SPE is a vector or matrix related to the sample dimension, while RMSE can...
PUM
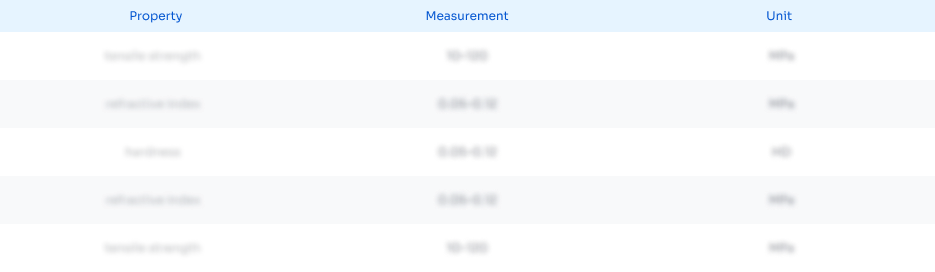
Abstract
Description
Claims
Application Information
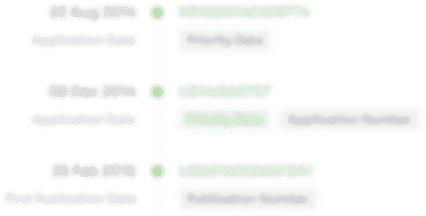
- R&D Engineer
- R&D Manager
- IP Professional
- Industry Leading Data Capabilities
- Powerful AI technology
- Patent DNA Extraction
Browse by: Latest US Patents, China's latest patents, Technical Efficacy Thesaurus, Application Domain, Technology Topic, Popular Technical Reports.
© 2024 PatSnap. All rights reserved.Legal|Privacy policy|Modern Slavery Act Transparency Statement|Sitemap|About US| Contact US: help@patsnap.com