Deep neural network compression method, system and device based on multi-group tensor decomposition and storage medium
A technology of deep neural network and compression method, applied in the fields of system, deep neural network compression method, device and storage medium, can solve the problems of large storage capacity, high computational complexity of deep neural network, difficult application of mobile devices, etc. Parameter ratio, the effect of reducing parameters
- Summary
- Abstract
- Description
- Claims
- Application Information
AI Technical Summary
Problems solved by technology
Method used
Image
Examples
Embodiment Construction
[0033] The invention discloses a deep neural network compression method based on multi-group tensor decomposition, in particular a set of low-rank and sparse compression models. We use TT decomposition for low-rank operations, and we retain the top 0.6 percent of the sparse structure with the largest absolute value. Adding sparsity in this way has little effect on the compression ratio. In addition, a Multi-TT structure is also constructed, which can well understand the characteristics of existing models and improve the accuracy of the model. Furthermore, the use of sparse structure is not important when using this method, and the Multi-TT structure can well explore the structure of the model.
[0034] 1. Symbols and definitions
[0035] First, the symbols and preparations of the present invention are defined. Scalars, vectors, matrices, and tensors are denoted by italic, bold lowercase, bold uppercase, and bold calligraphic symbols, respectively. This means that the dime...
PUM
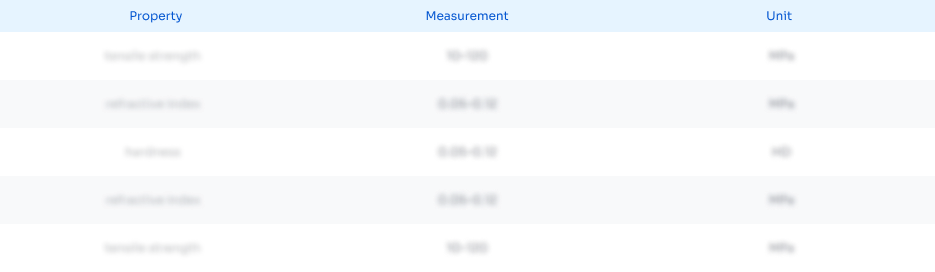
Abstract
Description
Claims
Application Information
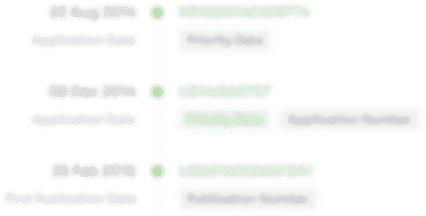
- R&D
- Intellectual Property
- Life Sciences
- Materials
- Tech Scout
- Unparalleled Data Quality
- Higher Quality Content
- 60% Fewer Hallucinations
Browse by: Latest US Patents, China's latest patents, Technical Efficacy Thesaurus, Application Domain, Technology Topic, Popular Technical Reports.
© 2025 PatSnap. All rights reserved.Legal|Privacy policy|Modern Slavery Act Transparency Statement|Sitemap|About US| Contact US: help@patsnap.com