A lesion classification system based on deep learning and probabilistic radiomics
A deep learning and radiomics technology, which is applied in the field of lesion classification system and lesion CT image classification, can solve the problems of insufficient classification accuracy, ambiguity of lesion classification, lack of controllability and transparency, etc.
- Summary
- Abstract
- Description
- Claims
- Application Information
AI Technical Summary
Problems solved by technology
Method used
Image
Examples
Embodiment 1
[0053] In this embodiment, the present invention proposes a lesion classification system for classifying lung CT images of pulmonary nodules. The scope of application of the present invention is not limited to pulmonary nodules, and is also applicable to the classification of other lesions (such as masses, etc.).
[0054] The overall framework of the lesion classification system based on deep learning and probabilistic radiomics proposed by the present invention is as follows: figure 1 shown, including:
[0055] Data collection module: using the public lung nodule dataset LIDC-IDRI (Armato SG III, et al.: The Lung Image Database Consortium (LIDC) and Image Database Resource Initiative (IDRI): A completed reference database of lung nodules on CT scans. MedicalPhysics, 38: 915--931, 2011), the public data set contains 2635 nodules, each of which is labeled by 4 experienced radiologists, and all 2635 nodules are identified by 4 doctors The same lesion is classified and segmente...
PUM
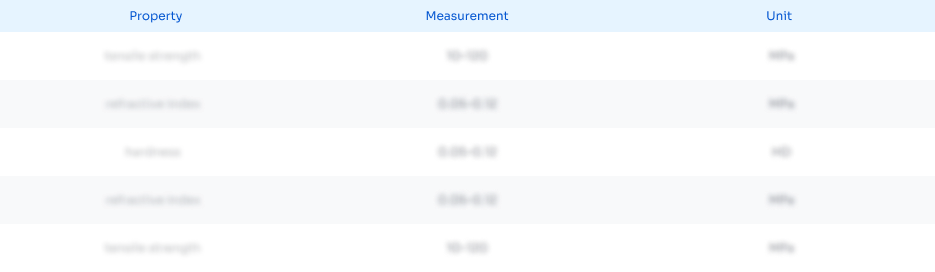
Abstract
Description
Claims
Application Information
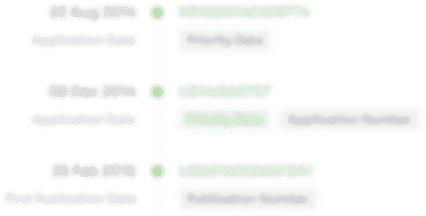
- R&D Engineer
- R&D Manager
- IP Professional
- Industry Leading Data Capabilities
- Powerful AI technology
- Patent DNA Extraction
Browse by: Latest US Patents, China's latest patents, Technical Efficacy Thesaurus, Application Domain, Technology Topic, Popular Technical Reports.
© 2024 PatSnap. All rights reserved.Legal|Privacy policy|Modern Slavery Act Transparency Statement|Sitemap|About US| Contact US: help@patsnap.com