Hazardous article image detection method, device, equipment and medium under articulated naturality web
A dangerous object and image detection technology, applied in the field of visual networking, can solve problems such as fatigue, waste of human resources, inspection omissions, etc., and achieve the effects of increasing robustness, reducing labor costs, and improving speed and accuracy
- Summary
- Abstract
- Description
- Claims
- Application Information
AI Technical Summary
Problems solved by technology
Method used
Image
Examples
Embodiment Construction
[0072] Exemplary embodiments of the present invention will be described in more detail below with reference to the accompanying drawings. Although exemplary embodiments of the present invention are shown in the drawings, it should be understood that the invention may be embodied in various forms and should not be limited to the embodiments set forth herein. Rather, these embodiments are provided for more thorough understanding of the present invention and to fully convey the scope of the present invention to those skilled in the art.
[0073] The Internet of Vision is an important milestone in the development of the network. It is a real-time network that can realize real-time transmission of high-definition video, and push many Internet applications to high-definition video, high-definition face-to-face.
[0074] The Internet of View adopts real-time high-definition video exchange technology, which can provide required services on one network platform, such as high-definition...
PUM
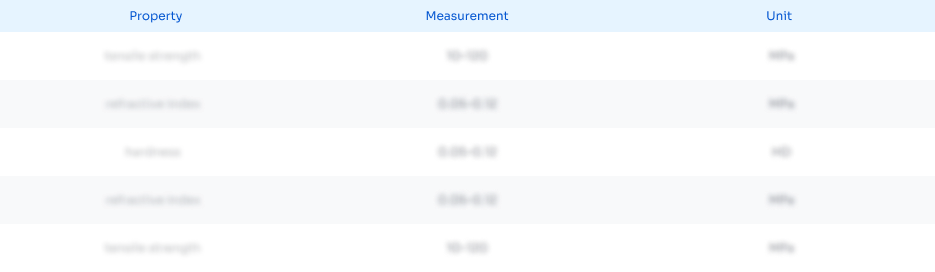
Abstract
Description
Claims
Application Information
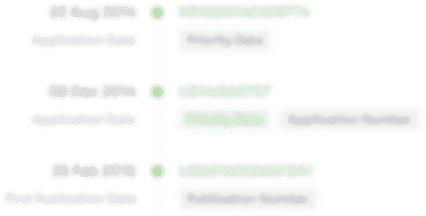
- R&D
- Intellectual Property
- Life Sciences
- Materials
- Tech Scout
- Unparalleled Data Quality
- Higher Quality Content
- 60% Fewer Hallucinations
Browse by: Latest US Patents, China's latest patents, Technical Efficacy Thesaurus, Application Domain, Technology Topic, Popular Technical Reports.
© 2025 PatSnap. All rights reserved.Legal|Privacy policy|Modern Slavery Act Transparency Statement|Sitemap|About US| Contact US: help@patsnap.com