Power quality disturbance identification method and system based on variable recurrent neural network, and medium
A cyclic neural network and power quality disturbance technology, applied in neural learning methods, biological neural network models, neural architectures, etc., can solve problems such as gradient exposure, probability influence, loss of randomness, etc., to reduce costs and enrich application scenarios , disturbance recognition speed and the effect of disturbance accuracy improvement
- Summary
- Abstract
- Description
- Claims
- Application Information
AI Technical Summary
Problems solved by technology
Method used
Image
Examples
Embodiment Construction
[0041] Such as figure 1 As shown, the implementation steps of the power quality disturbance identification method based on the variable cyclic neural network in this embodiment include:
[0042] 1) Input power quality disturbance waveform data;
[0043] 2) Perform feature extraction on the power quality disturbance waveform data to obtain multi-dimensional feature vectors;
[0044] 3) Input the multidimensional feature vector into the pre-trained variable cyclic neural network, which includes a random layer for introducing potential random variables to the output layer, and is pre-trained to establish the multidimensional eigenvector and its The mapping relationship between the output probabilities corresponding to N kinds of disturbances;
[0045] 4) The output of the variable recurrent neural network is generated through an activation function to generate an output probability curve corresponding to N kinds of disturbances;
[0046] 5) Determine the disturbance type at a ...
PUM
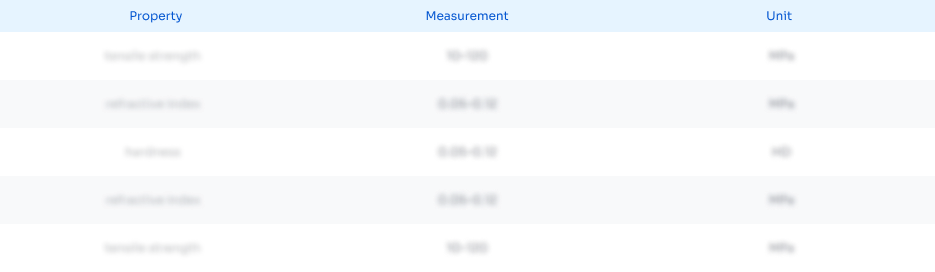
Abstract
Description
Claims
Application Information
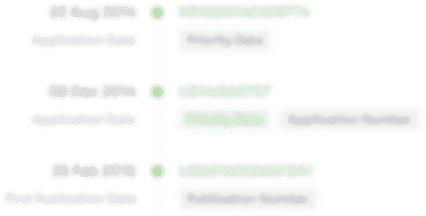
- R&D
- Intellectual Property
- Life Sciences
- Materials
- Tech Scout
- Unparalleled Data Quality
- Higher Quality Content
- 60% Fewer Hallucinations
Browse by: Latest US Patents, China's latest patents, Technical Efficacy Thesaurus, Application Domain, Technology Topic, Popular Technical Reports.
© 2025 PatSnap. All rights reserved.Legal|Privacy policy|Modern Slavery Act Transparency Statement|Sitemap|About US| Contact US: help@patsnap.com