Rapid image style migration method based on group normalization
A normalization and style technology, applied in the field of image processing, can solve the problems of slow model convergence, fuzziness, constraints, etc., and achieve the effects of faster convergence, enhanced learning, and improved performance
- Summary
- Abstract
- Description
- Claims
- Application Information
AI Technical Summary
Problems solved by technology
Method used
Image
Examples
Embodiment 1
[0038] The invention provides a real-time fast image style transfer method, such as figure 1 As shown, the present invention adopts a framework structure combining generator network and loss network, and the specific steps include:
[0039] (1) The size of the input image is transformed to 256*256, which is convenient for training the generator network.
[0040] (2). Start adding extra borders on the top, bottom, left, and right of the input image to eliminate edge effects.
[0041] (3) Combination figure 2 As shown, the input content image x is input into the generator network, and converted into an output image y through y=fw(x) mapping.
[0042] (4) Eliminate the extra border that was added to prevent edge effects at the beginning, and get the final output image y k .
[0043] (5), the image y output through the generator network k And the original content image y c Input to the pre-trained vgg16 network model.
[0044] (6) Use image y k The feature map and original content image y ...
Embodiment 2
[0065] The present invention also provides a fast image style transfer method that uses group normalization instead of batch normalization and instance normalization in the generator network. The specific steps include:
[0066] (1) The size of the input image is transformed to 256*256, which is convenient for training the generator network.
[0067] (2). Start adding extra borders on the top, bottom, left, and right of the input image to eliminate edge effects.
[0068] (3) Input the input image x into the generator network. The generator network is set as a deep residual network, and the network structure is two down-sampling convolutional layers, five residual units, two up-sampling deconvolution layers, and finally an output layer. The main principle of the generator network is to perform convolution calculation and deconvolution calculation on the input image. Using down-sampling convolutional layer and up-sampling deconvolutional layer can reduce the complexity of calculation...
PUM
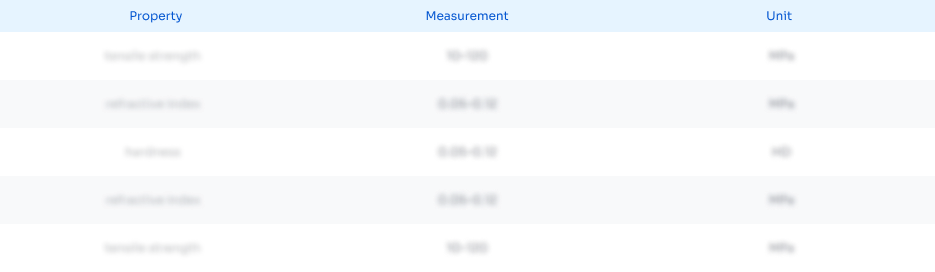
Abstract
Description
Claims
Application Information
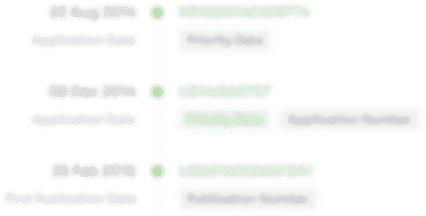
- R&D Engineer
- R&D Manager
- IP Professional
- Industry Leading Data Capabilities
- Powerful AI technology
- Patent DNA Extraction
Browse by: Latest US Patents, China's latest patents, Technical Efficacy Thesaurus, Application Domain, Technology Topic, Popular Technical Reports.
© 2024 PatSnap. All rights reserved.Legal|Privacy policy|Modern Slavery Act Transparency Statement|Sitemap|About US| Contact US: help@patsnap.com