Shadow rough fuzzy clustering method based on Mahalanobis distance
A fuzzy clustering method and Mahalanobis distance technology, applied in the field of data processing, can solve the problems of abnormal uncertainty of data, unsatisfactory division of boundary areas, etc., and achieve the effect of improving the uneven distribution of samples and effective cluster division.
- Summary
- Abstract
- Description
- Claims
- Application Information
AI Technical Summary
Problems solved by technology
Method used
Image
Examples
example
[0132] There are 4 UCI datasets, 9-dimensional Glass dataset, 13-dimensional Wine dataset, 8-dimensional Pima dataset and 13-dimensional Heart disease dataset. The Glass dataset has a total of 214 samples with 6 clusters of 70, 76, 17, 13, 9 and 29 samples. The Wine dataset has a total of 178 samples and has 3 clusters with 59, 71 and 48 samples respectively. The Pima dataset contains 768 samples and has 2 clusters with 500 and 268 samples respectively. The Heart disease dataset has a total of 270 samples and has 2 clusters with 150 and 120 samples respectively. Simulation experiments are carried out on the real data sets Glass, Wine, Pima and Heart disease. The comparison of the misclassification rates of the four algorithms in the real data set clustering is shown in Table 2.
[0133] Table 2
[0134]
[0135]
[0136] After analysis, MSRFCM algorithm has the lowest misclassification rate compared with MFCM and FCM algorithms on 4 real data sets. The MFCM algorith...
PUM
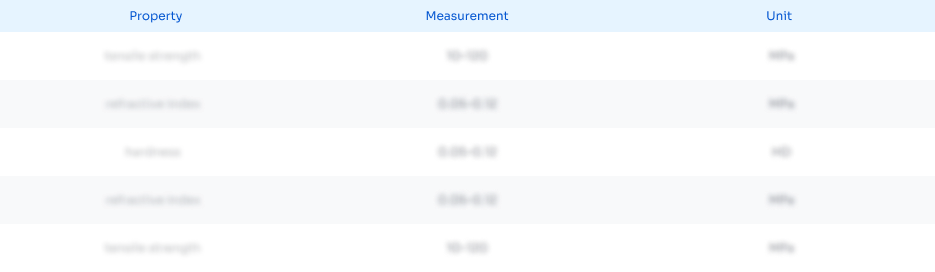
Abstract
Description
Claims
Application Information
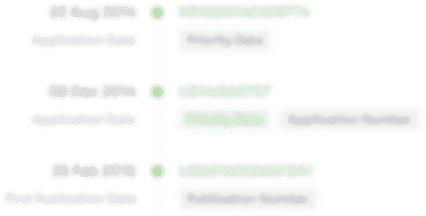
- R&D
- Intellectual Property
- Life Sciences
- Materials
- Tech Scout
- Unparalleled Data Quality
- Higher Quality Content
- 60% Fewer Hallucinations
Browse by: Latest US Patents, China's latest patents, Technical Efficacy Thesaurus, Application Domain, Technology Topic, Popular Technical Reports.
© 2025 PatSnap. All rights reserved.Legal|Privacy policy|Modern Slavery Act Transparency Statement|Sitemap|About US| Contact US: help@patsnap.com