Hybrid recommendation method based on graph convolutional neural network
A convolutional neural network and mixed recommendation technology, applied in neural learning methods, biological neural network models, instruments, etc., can solve the problems of traditional methods such as data sparseness, heavy feature engineering, and failure to satisfy users, so as to improve accuracy and diversity performance, alleviate heavy feature engineering, and alleviate the effect of data sparsity
- Summary
- Abstract
- Description
- Claims
- Application Information
AI Technical Summary
Problems solved by technology
Method used
Image
Examples
Embodiment Construction
[0031] In order to describe the present invention more specifically, the technical solutions of the present invention will be described in detail below in conjunction with the accompanying drawings and specific embodiments.
[0032] The hybrid recommendation method based on graph convolutional neural network of the present invention comprises the following steps:
[0033] (1). Collect behavioral data of all users U={u 1 , u 2 ,...,u |U|} is the set of all users, where the behavior data of user u∈U is expressed as a sequence Bu={(i 1 ,t 1 ),(i 2 ,t 2 ),…,(i m ,t m )}, (i j ,t j ) represent the items interacted by the user and the interaction time respectively, I={i 1 ,i 2 ,...,i |M|} is the collection of all items. Collect attribute data A of all items in I, including but not limited to categories, tags, metadata and other information.
[0034] (2). Construct a heterogeneous information graph G=(N,E) based on the behavior data of all users and the attribute data...
PUM
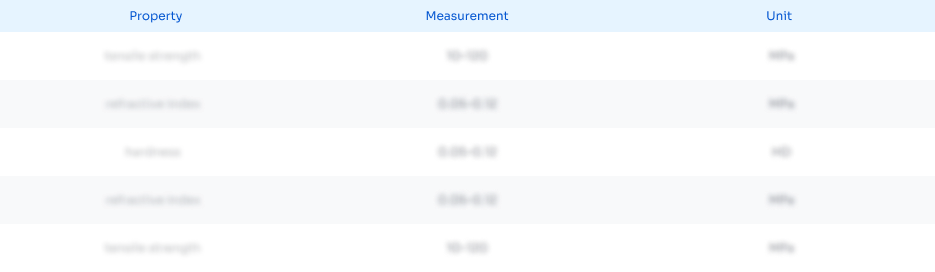
Abstract
Description
Claims
Application Information
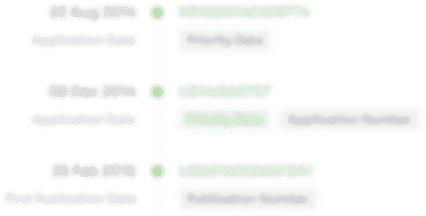
- R&D
- Intellectual Property
- Life Sciences
- Materials
- Tech Scout
- Unparalleled Data Quality
- Higher Quality Content
- 60% Fewer Hallucinations
Browse by: Latest US Patents, China's latest patents, Technical Efficacy Thesaurus, Application Domain, Technology Topic, Popular Technical Reports.
© 2025 PatSnap. All rights reserved.Legal|Privacy policy|Modern Slavery Act Transparency Statement|Sitemap|About US| Contact US: help@patsnap.com