Grayscale image colorization method based on convolutional neural network
A convolutional neural network and grayscale image technology, applied in the field of grayscale image colorization, can solve the problems of insufficient image feature extraction, few layers, and loss of local information in the image, and achieve good colorization effect and fewer layers Number of applications, large effect of the scene
- Summary
- Abstract
- Description
- Claims
- Application Information
AI Technical Summary
Problems solved by technology
Method used
Image
Examples
Embodiment Construction
[0036] In order to further understand the invention content, characteristics and effects of the present invention, the following embodiments are enumerated hereby, and detailed descriptions are as follows in conjunction with the accompanying drawings:
[0037] See Figure 1 to Figure 4 , a method for colorizing grayscale images based on convolutional neural networks, a convolutional neural network is established, and grayscale images are converted into color images; the hidden layer of the convolutional neural network includes multiple connection layers, each connection layer Including a sequentially connected convolution layer, a batch normalization layer and a combined nonlinear activation function layer; the combined nonlinear activation function layer includes a sequentially connected nonlinear activation function layer, a single-channel convolution kernel layer, a batch normalization layer and Normalization layer: the combined non-linear activation function layer performs...
PUM
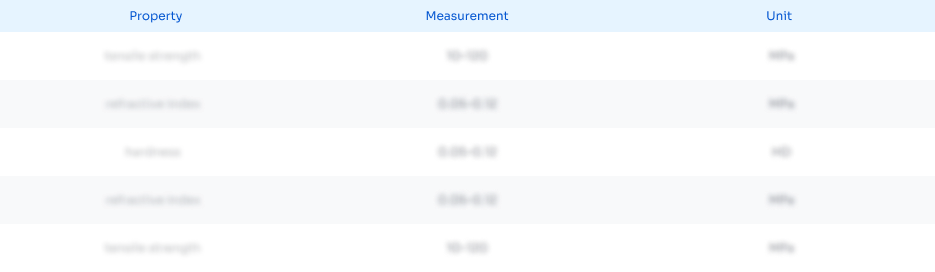
Abstract
Description
Claims
Application Information
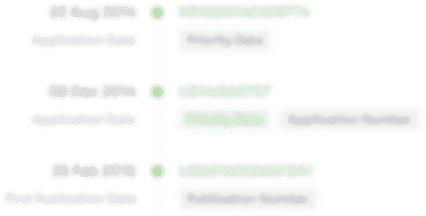
- R&D Engineer
- R&D Manager
- IP Professional
- Industry Leading Data Capabilities
- Powerful AI technology
- Patent DNA Extraction
Browse by: Latest US Patents, China's latest patents, Technical Efficacy Thesaurus, Application Domain, Technology Topic, Popular Technical Reports.
© 2024 PatSnap. All rights reserved.Legal|Privacy policy|Modern Slavery Act Transparency Statement|Sitemap|About US| Contact US: help@patsnap.com