Protein-ligand binding site prediction algorithm based on deep learning
A technology of ligand binding site and prediction algorithm, applied in the fields of protein biology and pattern recognition, can solve the problem of low accuracy of prediction algorithm, and achieve the effect of high prediction accuracy
- Summary
- Abstract
- Description
- Claims
- Application Information
AI Technical Summary
Problems solved by technology
Method used
Image
Examples
Embodiment
[0092] protein and MN 2+ The binding site data set is used as training set and test set. The training set contains a total of 440 proteins, including 1931 binding residues and 150229 non-binding residues; the test set contains 144 proteins, including 612 binding residues and 50838 non-binding residues.
[0093] First, the PSI-BLAST algorithm, HHblits algorithm, SCRATCH algorithm and S-SITE algorithm were used to extract the evolution information, secondary structure information, relative solvent accessibility and binding probability of all proteins in the training set and test set, respectively, and the evolution information ( Including PSSM and HHM) for normalization processing; secondly, calculate the Euclidean distance between each residue pair from the three-dimensional space coordinates of each residue of the protein in all training sets and test sets, and construct a distance matrix, and matrix The number of columns is scaled to 400; finally, the feature tensor is inter...
PUM
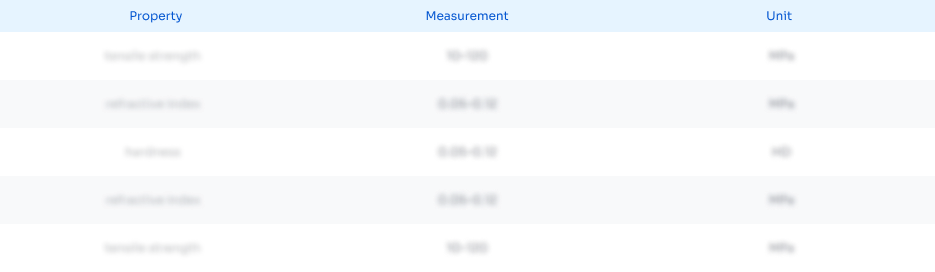
Abstract
Description
Claims
Application Information
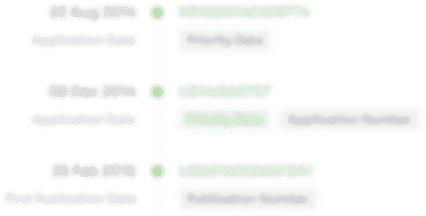
- R&D Engineer
- R&D Manager
- IP Professional
- Industry Leading Data Capabilities
- Powerful AI technology
- Patent DNA Extraction
Browse by: Latest US Patents, China's latest patents, Technical Efficacy Thesaurus, Application Domain, Technology Topic, Popular Technical Reports.
© 2024 PatSnap. All rights reserved.Legal|Privacy policy|Modern Slavery Act Transparency Statement|Sitemap|About US| Contact US: help@patsnap.com