Image super-resolution method based on hierarchical residual neural network
A neural network and super-resolution technology, applied in the field of image super-resolution based on hierarchical residual neural network, can solve the problems of lack of efficient network model, performance can not meet practical application requirements, etc., to improve reconstruction effect, improve Forward propagation and back propagation, the effect of small network model parameters
- Summary
- Abstract
- Description
- Claims
- Application Information
AI Technical Summary
Problems solved by technology
Method used
Image
Examples
Embodiment Construction
[0018] This embodiment provides an image super-resolution method based on a hierarchical residual neural network, which involves three parts: network structure, image preprocessing, and implementation details. This method uses a hierarchical residual learning strategy and a dual-domain enhancement module to construct a hierarchical residual neural network model, which is used to learn the complex mapping relationship between low-resolution and high-resolution images, and then utilizes the trained network The model reconstructs a high-resolution image from an input low-resolution image.
[0019] figure 1 It is the network structure diagram of the image super-resolution reconstruction model, which is composed of feature extraction layer, feature mapping layer and feature fusion layer. The feature extraction layer is composed of convolution (Conv) module, and the feature mapping layer is composed of multiple dual-domain enhancement modules ( DEM) cascade structure, the feature f...
PUM
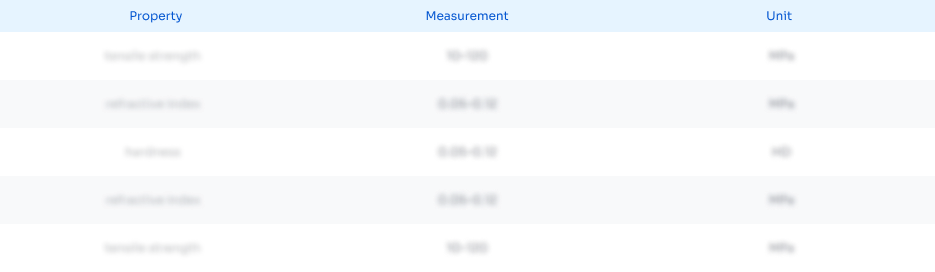
Abstract
Description
Claims
Application Information
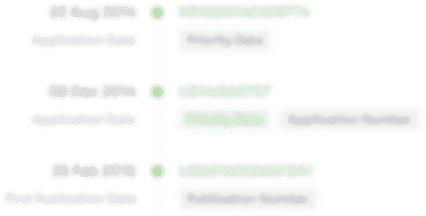
- R&D Engineer
- R&D Manager
- IP Professional
- Industry Leading Data Capabilities
- Powerful AI technology
- Patent DNA Extraction
Browse by: Latest US Patents, China's latest patents, Technical Efficacy Thesaurus, Application Domain, Technology Topic, Popular Technical Reports.
© 2024 PatSnap. All rights reserved.Legal|Privacy policy|Modern Slavery Act Transparency Statement|Sitemap|About US| Contact US: help@patsnap.com