Unsupervised relation prediction method based on depth map network auto-encoder
A technology of self-encoder and prediction method, applied in the field of big data analysis, can solve the problems of inability to use node attributes, lower statistical validity, lower efficiency, etc.
- Summary
- Abstract
- Description
- Claims
- Application Information
AI Technical Summary
Problems solved by technology
Method used
Image
Examples
example
[0110] Step 1: First, download the gene pair (entity pair) data set file and the gene (entity) file contained in the data set that constitute collaborative lethality (that is, the target relationship is collaborative lethality) from a relational database, involving 6375 entities, a total of 19677 Entity pairs known to form the target relationship. Part of the entity pair dataset is shown in Table 2:
[0111] Parts of the entity dataset files involved are shown in Table 1:
[0112] A2M A2ML1 AADAT AAR2 AATF
[0113] Table 1
[0114] The two character strings in each line (19667 lines in total) in the file represent two entities that can form the target relationship. In order to make the description of the following steps more concise and easy to understand, when describing the preprocessing part, only the changes of the first five entity pairs are included:
[0115] BTG2 SESN1 EGR1 FOSB MYOF PINK1 DNAJB6 GLU...
PUM
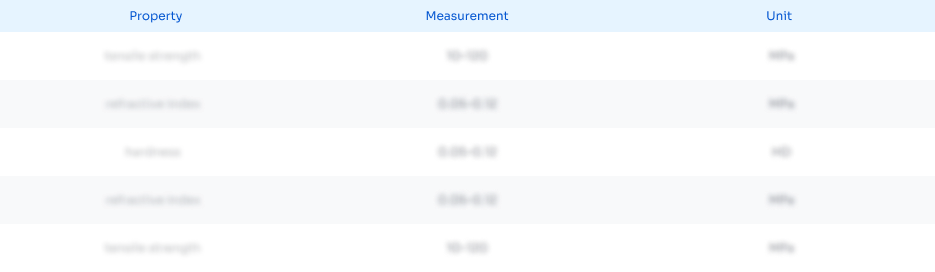
Abstract
Description
Claims
Application Information
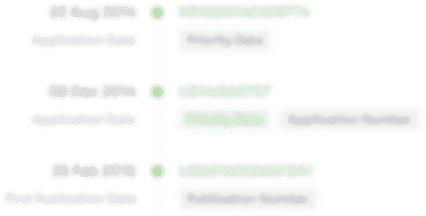
- R&D
- Intellectual Property
- Life Sciences
- Materials
- Tech Scout
- Unparalleled Data Quality
- Higher Quality Content
- 60% Fewer Hallucinations
Browse by: Latest US Patents, China's latest patents, Technical Efficacy Thesaurus, Application Domain, Technology Topic, Popular Technical Reports.
© 2025 PatSnap. All rights reserved.Legal|Privacy policy|Modern Slavery Act Transparency Statement|Sitemap|About US| Contact US: help@patsnap.com