Edge computing privacy protection system and method based on joint learning
A privacy protection system and edge computing technology, applied in the fields of data encryption, deep learning, differential privacy, and distributed computing, can solve problems such as high communication costs, damage to the accuracy of the global model, and cumulative noise to ensure authenticity and solve problems Effects of Conflicting Problems on Privacy Preservation and Learning Accuracy
- Summary
- Abstract
- Description
- Claims
- Application Information
AI Technical Summary
Problems solved by technology
Method used
Image
Examples
Embodiment
[0053] Step 1: Perturb the parameters on the client side.
[0054] We consider letting users add perturbations to their private data themselves. Depending on the discrete groups used in the encryption scheme, we use the geometric distribution (Geo), which can be viewed as a discrete approximation of the Laplace distribution. For any integer z, its probability mass function is:
[0055]
[0056] where the parameter α∈(0,1).
[0057] We can construct DD-Private data perturbations simply by adding Geo(α) to each participant’s raw data:
[0058]
[0059] in, is a scaling function of the sensitivity coefficient S, and the above definition means that if Geo(α) noise is incorporated into the user's data, α-DP will be achieved.
[0060] Step 2: Encrypt the data on the client side.
[0061] There is a trusted authority (trusted authority, TA) in our PPFL system, and this trusted authority can send keys to clients and servers. TA only participates in the initialization phas...
PUM
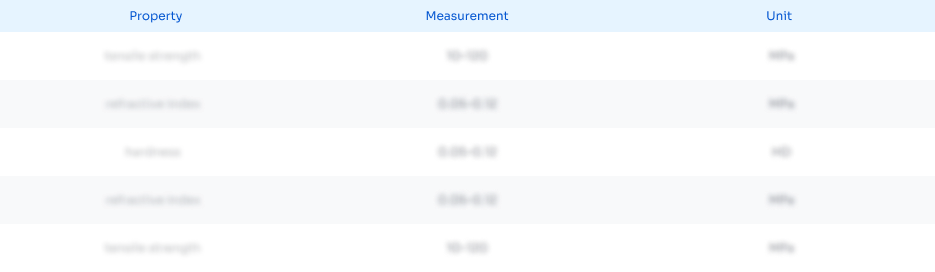
Abstract
Description
Claims
Application Information
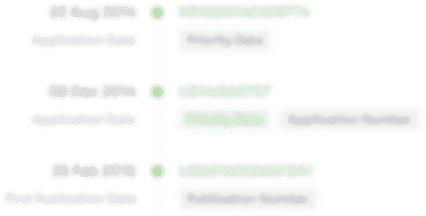
- R&D Engineer
- R&D Manager
- IP Professional
- Industry Leading Data Capabilities
- Powerful AI technology
- Patent DNA Extraction
Browse by: Latest US Patents, China's latest patents, Technical Efficacy Thesaurus, Application Domain, Technology Topic, Popular Technical Reports.
© 2024 PatSnap. All rights reserved.Legal|Privacy policy|Modern Slavery Act Transparency Statement|Sitemap|About US| Contact US: help@patsnap.com