Problem classification method and system based on multi-attention mechanism and storage medium
A problem classification and attention technology, applied in neural learning methods, text database clustering/classification, computer parts, etc., can solve the problem of a single convolutional neural network long and short-term memory network, no problem text extraction, no further mining problems Issues such as textual underlying topic information
- Summary
- Abstract
- Description
- Claims
- Application Information
AI Technical Summary
Problems solved by technology
Method used
Image
Examples
Embodiment
[0139] The following three data sets are used to test the technical effects of five problem classification models in the prior art and the method provided by the present invention, wherein the three data sets include:
[0140] 1. The data set provided by Baidu Lab, which includes 6205 pieces of data, that is, 6205 questions and corresponding answers, such as: Who is the author of the book "Basics of Mechanical Design"? The corresponding answers are: Yang Kezhen, Cheng Guangyun, Li Zhong;
[0141] 2. The public question set of the China Computer Federation (CCF) 2016 International Conference on Natural Language Processing and Chinese Computing Questions and Answers (hereinafter referred to as the data set NLPCC2016), which contains 9604 pieces of data, such as: Lu Xun's "Chaohua How many characters are there in the book Xi Shi? The corresponding answer is: 100 thousand words;
[0142] 3. The public question set of the CCF 2017 International Conference on Natural Language Proc...
PUM
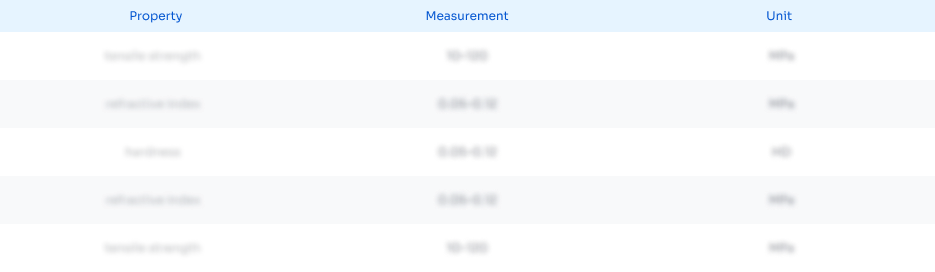
Abstract
Description
Claims
Application Information
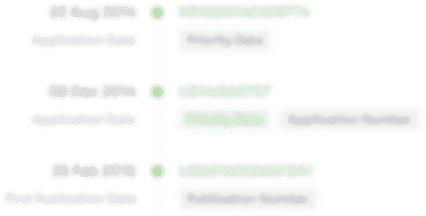
- R&D
- Intellectual Property
- Life Sciences
- Materials
- Tech Scout
- Unparalleled Data Quality
- Higher Quality Content
- 60% Fewer Hallucinations
Browse by: Latest US Patents, China's latest patents, Technical Efficacy Thesaurus, Application Domain, Technology Topic, Popular Technical Reports.
© 2025 PatSnap. All rights reserved.Legal|Privacy policy|Modern Slavery Act Transparency Statement|Sitemap|About US| Contact US: help@patsnap.com