Critical heat flux density prediction method based on deep learning support vector machine
A technology of critical heat flux and support vector machine, applied in forecasting, computer components, instruments, etc., can solve problems such as predicting critical heat flux, achieve the effect of improving prediction ability, avoiding uncertainty, and speeding up training
- Summary
- Abstract
- Description
- Claims
- Application Information
AI Technical Summary
Problems solved by technology
Method used
Image
Examples
Embodiment Construction
[0044] The present invention will be described in detail below in conjunction with the accompanying drawings and specific embodiments.
[0045] The present invention is based on the critical heat flux prediction method of deep learning support vector machine, specifically implements according to the following steps:
[0046] Step 1: The collected critical heat flux raw data is divided into two parts: 70% of the raw data is used as a training set, and X={(x 1 ,y 1 ),(x 2 ,y 2 ),…(x i ,y i )...(x n ,y n )} means that for x in the obtained training data set i Use linear transformation for normalization processing to obtain the normalized data point x′ i ;Another 30% of the original data is used as a test set to test the prediction accuracy of the trained prediction model;
[0047] where x i For the system pressure P, mass flow rate G and equilibrium steam content X e Composed of i-th vector data points, i=1,2,...,n; x' i for x i Normalized data points; y i means x ...
PUM
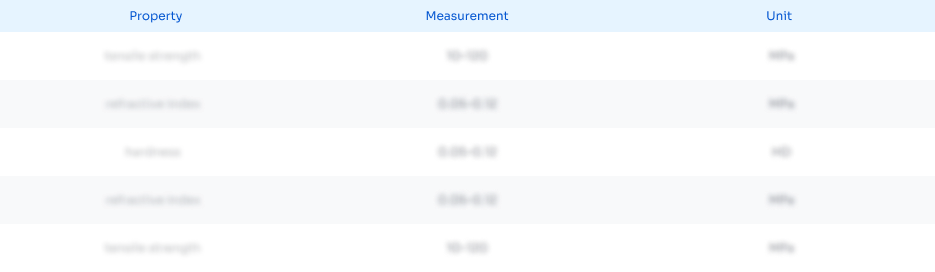
Abstract
Description
Claims
Application Information
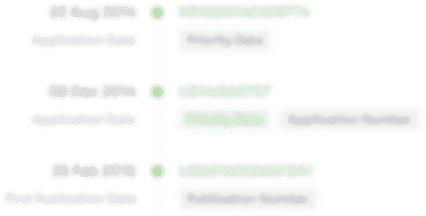
- R&D
- Intellectual Property
- Life Sciences
- Materials
- Tech Scout
- Unparalleled Data Quality
- Higher Quality Content
- 60% Fewer Hallucinations
Browse by: Latest US Patents, China's latest patents, Technical Efficacy Thesaurus, Application Domain, Technology Topic, Popular Technical Reports.
© 2025 PatSnap. All rights reserved.Legal|Privacy policy|Modern Slavery Act Transparency Statement|Sitemap|About US| Contact US: help@patsnap.com