LSTM neural network training method and device
A neural network training and neural network technology, which is applied in the field of LSTM neural network training methods and devices, can solve the problems of high training calculation overhead and complex Transformer model structure, and achieve the effects of improving carrying capacity, increasing calculation amount, and improving data quality.
- Summary
- Abstract
- Description
- Claims
- Application Information
AI Technical Summary
Problems solved by technology
Method used
Image
Examples
Embodiment 1
[0041] A LSTM neural network training method, including training data generated by unlabeled text, after processing keywords in the unlabeled text, weighting the training data according to the keywords, improving the ability of the training data to carry feature information, and weighting the The training data is used for LSTM neural network training. The present invention draws on the physiological basis that human beings focus on key positions or words when acquiring information, and combines the long-short-term memory network LSTM to propose a model training method that does not change the model structure, by changing the weight of key information in the training data , to obtain better model training results.
Embodiment 2
[0043] The difference between this embodiment and Embodiment 1 is that the training data generated by the unlabeled text processes the keywords in the unlabeled text and then weights the training data according to the keywords, so as to improve the carrying capacity of the training data for feature information , the method of using weighted training data for LSTM neural network training includes the following steps:
[0044] S1. Taking the unlabeled text as the training text, and preprocessing the training text;
[0045] S2. Identify the preprocessed training text and generate keywords of the training text;
[0046] S3. Encoding the words in the training text to obtain high-dimensional space continuous word vectors, and performing the same encoding to keywords to obtain keyword vectors;
[0047] S4. Add the keyword vector to the corresponding word vector to weight the word vector to obtain the final training data;
[0048] S5. Input the final training data into the LSTM neur...
Embodiment 3
[0050] The difference between this embodiment and Embodiment 2 is that the method for preprocessing the training text in step S1 includes at least one of cleaning, word segmentation, and removing stop words.
[0051] Further, the keywords in the step S2 include entity keywords, relationship keywords and event keywords. Perform named entity recognition on the preprocessed training text, obtain common named entities such as name, address, organization, time, currency, quantity, etc., and establish entity keywords. Then extract the entity relationship from the preprocessing training text. If there is a relationship between entities, judge whether the entity relationship belongs to common components and wholes, tool usage, member set, cause and effect, entity destination, content and container, information and theme, production Types such as Produced and Entity and Origin, and form relational keywords. Event extraction is performed on the pre-processing training text. If there is...
PUM
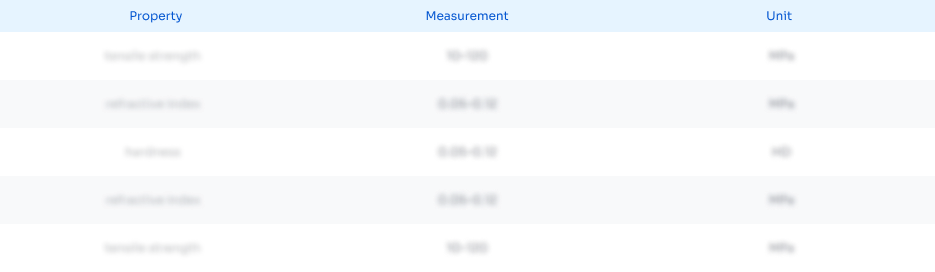
Abstract
Description
Claims
Application Information
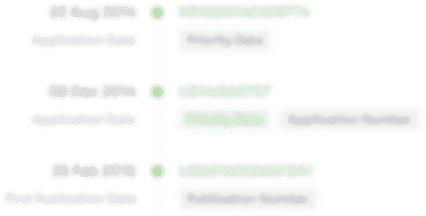
- Generate Ideas
- Intellectual Property
- Life Sciences
- Materials
- Tech Scout
- Unparalleled Data Quality
- Higher Quality Content
- 60% Fewer Hallucinations
Browse by: Latest US Patents, China's latest patents, Technical Efficacy Thesaurus, Application Domain, Technology Topic, Popular Technical Reports.
© 2025 PatSnap. All rights reserved.Legal|Privacy policy|Modern Slavery Act Transparency Statement|Sitemap|About US| Contact US: help@patsnap.com