Hybrid wind power prediction method based on long-short-term memory neural network
A long-short-term memory, neural network technology, applied in the field of hybrid wind power prediction based on long-short-term memory neural network, can solve the problems of not considering time-dependent information, high computational cost, affecting algorithm learning efficiency, etc.
- Summary
- Abstract
- Description
- Claims
- Application Information
AI Technical Summary
Problems solved by technology
Method used
Image
Examples
Embodiment Construction
[0058] The specific implementation manners of the present invention will be further described in detail below in conjunction with the accompanying drawings. The following examples are used to illustrate the present invention, but are not intended to limit the scope of the present invention.
[0059] Such as figure 1 As shown, the method of this embodiment is as follows.
[0060] Step 1: Select historical wind speed (instantaneous wind speed, average wind speed in the past 30 seconds, average wind speed in the past 10 minutes), active power data (from the previous 1 moment to the previous 20 moments) and grid phase voltage (phase A, B phase, C phase), generator speed, wind rotor speed, gearbox oil temperature, a total of 29 kinds of characteristic attributes as alternative input variables As shown in Table 1;
[0061] Table 1 Wind power related variable table
[0062]
[0063]
[0064] Step 1-1: Using the decision tree algorithm, calculate a single input variable F...
PUM
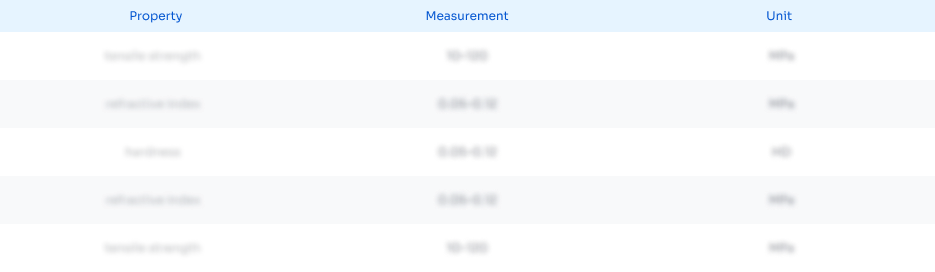
Abstract
Description
Claims
Application Information
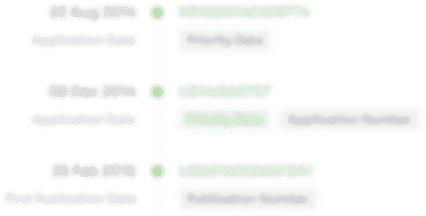
- R&D
- Intellectual Property
- Life Sciences
- Materials
- Tech Scout
- Unparalleled Data Quality
- Higher Quality Content
- 60% Fewer Hallucinations
Browse by: Latest US Patents, China's latest patents, Technical Efficacy Thesaurus, Application Domain, Technology Topic, Popular Technical Reports.
© 2025 PatSnap. All rights reserved.Legal|Privacy policy|Modern Slavery Act Transparency Statement|Sitemap|About US| Contact US: help@patsnap.com