Method for monitoring and evaluating actual state of MOV based on outlier detection
An actual state, outlier technology, applied in the direction of measuring electricity, measuring devices, measuring electrical variables, etc., can solve problems such as affecting the quality of the final solution, and achieve the effect of reducing the difficulty of execution, strengthening effective methods, and strengthening monitoring and maintenance.
- Summary
- Abstract
- Description
- Claims
- Application Information
AI Technical Summary
Problems solved by technology
Method used
Image
Examples
Embodiment Construction
[0047] The present invention will be described in further detail below in conjunction with the accompanying drawings.
[0048] Local Outlier Factor (LOF) is an efficient method based on performing outlier detection on moderately high-dimensional datasets. Its main purpose is to detect abnormal data or behaviors that are quite different from normal data behaviors or characteristic attributes. The algorithm reflects the degree of abnormality of a set of sample data based on the characteristic parameter of "local reachable density". If the local reachable density of this set of data points is higher, the point is more likely to be an outlier. For the monitoring and evaluation of the actual state of MOV, it means that this method can be used to combine a large number of MOV multi-parameter sampling data to train a model to learn the distribution of normal parameter data, and use this learned distribution to detect the data that cannot be detected. The outlier parameter data fit to ...
PUM
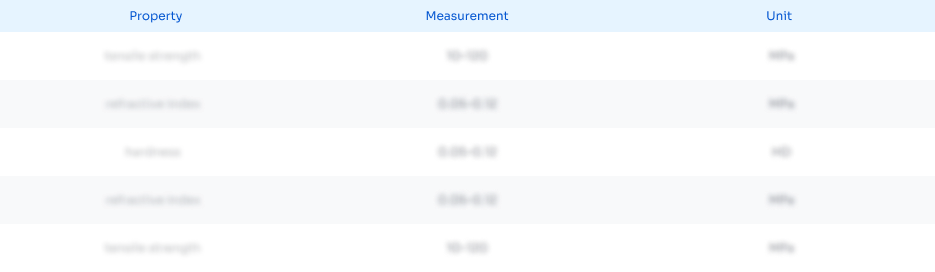
Abstract
Description
Claims
Application Information
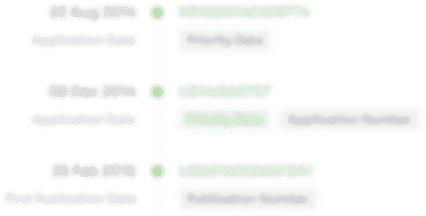
- R&D Engineer
- R&D Manager
- IP Professional
- Industry Leading Data Capabilities
- Powerful AI technology
- Patent DNA Extraction
Browse by: Latest US Patents, China's latest patents, Technical Efficacy Thesaurus, Application Domain, Technology Topic, Popular Technical Reports.
© 2024 PatSnap. All rights reserved.Legal|Privacy policy|Modern Slavery Act Transparency Statement|Sitemap|About US| Contact US: help@patsnap.com