Federated learning income distribution method and system
A distribution method and distribution system technology, applied in the field of income distribution mechanism in the federated learning process, can solve problems that cannot be directly applied in the federated learning field, and achieve the effect of process modularization and easy income and expenditure adjustment
- Summary
- Abstract
- Description
- Claims
- Application Information
AI Technical Summary
Problems solved by technology
Method used
Image
Examples
Embodiment 1
[0060] combine Figure 1-12 Shown: a federated learning income distribution method, the steps include:
[0061] Step 1: Assess the validity of the data contributed by each data provider in the federation;
[0062] Step 2: Measure the type parameters of federated learning participants;
[0063] Step 3: Calculate the pricing of the federated learning model;
[0064] Step 4: calculating the payment for each data provider based on one or more of the validity of the data contributed by each data provider, the type parameters of the federated learning participants, and the pricing of the federated learning model;
[0065] Optional step 5: Federated learning participants report their type parameters to the federated system;
[0066] Optional Step 6: Select an optimization target;
[0067] Optional Step 7: Calculate the dynamic financing rate of the federated learning system.
[0068] The federated learning participants include data providers and model users.
[0069] The data v...
Embodiment 2
[0091] combine figure 2 As shown, Step 1: Measure the validity of the data contributed by each data provider in the federation; the validity of a set of data is a measure of the set of data, and training with data with high data validity can result in a more efficient High-quality federated learning models. Data validity is obtained by measuring one or more of data size, data quality, number of features possessed, specificity of features possessed, and contribution to model quality. The size of the data is measured by the total number of records, which is the total number of all samples. The larger the data size, the higher the quality of the trained model, and the higher the data validity score given by the system. Data quality is measured by accuracy, that is, the ratio of the number of abnormal data records to the total number of records. The higher the ratio, the higher the impact of wrong data, and the lower the data validity score given by the system. Abnormal data i...
Embodiment 3
[0162] Corresponding to the second step of embodiment, see Figure 12 As shown, the system of the present invention includes the following modules.
[0163] Module 1: data validity measurement module, used to realize step 1.
[0164]Sub-module 1.1: a communication module, used to realize sub-step 1.1. In some implementations, the communication module supports the data provider to download the data validity measurement tool provided by the federated learning system; in other implementations, the communication module supports the data provider to encrypt and upload the data to the server installed with the measurement data validity tool.
[0165] Sub-module 1.2: data validity correlation coefficient measurement module, used to realize sub-step 1.2. In some implementations, the data validity correlation coefficient measurement module provides information on one or more of data quality, data size, number of features owned, specificity of features owned, contribution to model qua...
PUM
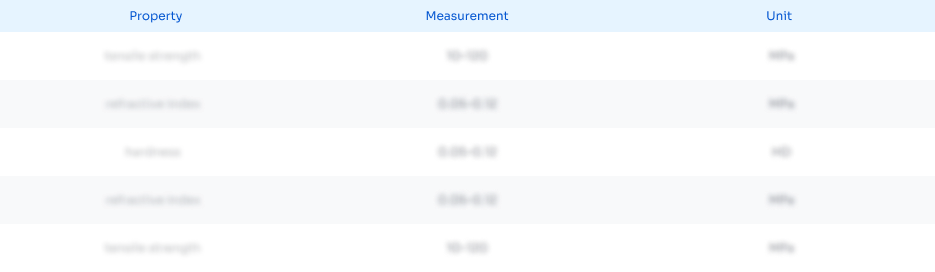
Abstract
Description
Claims
Application Information
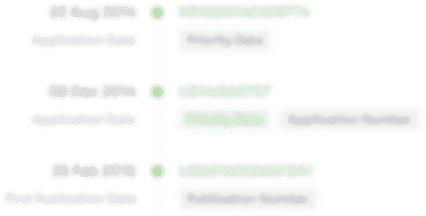
- R&D Engineer
- R&D Manager
- IP Professional
- Industry Leading Data Capabilities
- Powerful AI technology
- Patent DNA Extraction
Browse by: Latest US Patents, China's latest patents, Technical Efficacy Thesaurus, Application Domain, Technology Topic, Popular Technical Reports.
© 2024 PatSnap. All rights reserved.Legal|Privacy policy|Modern Slavery Act Transparency Statement|Sitemap|About US| Contact US: help@patsnap.com