Locust optimization-based situation analysis method and system for LSSVM-ARIMA model, and storage medium
An analysis method, locust technology, applied in the field of machine learning and data mining, can solve the problems of increased calculation, easy to fall into local optimum, increased model training time, etc., and achieve the effect of accurate situation prediction
- Summary
- Abstract
- Description
- Claims
- Application Information
AI Technical Summary
Problems solved by technology
Method used
Image
Examples
Embodiment 1
[0059] Taking the safety production data of all enterprises in Beijing as an example, the selected data include the implementation of the safety production responsibility system, the elimination of hidden dangers, and the ability to prevent and control accidents. There are a total of 3.22 million data records, including 3.11 million hidden danger data. Will image 3 The index data shown is used as the original time series data set, part of which is marked as a training set and part of which is marked as a test set, with a total of 2.21 million training sample data and 900,000 test sample data. Firstly, the locust optimization algorithm is used to optimize the parameters of the LSSVM model globally, and the optimal solution of the parameters is found, so as to construct the LSSVM model; secondly, the training samples and test samples are divided into low-frequency components and high-frequency components by using the wavelet decomposition algorithm; then, using The high-frequen...
Embodiment 2
[0099] This embodiment provides a readable storage medium, in which program instructions are stored, and the computer executes the situation analysis method based on the locust-optimized LSSVM-ARIMA model described in Embodiment 1 after reading the program instructions.
Embodiment 3
[0101] This embodiment provides a situation analysis system based on the locust-optimized LSSVM-ARIMA model, including at least one processor and at least one memory, at least one of the memory stores program instructions, and at least one processor reads the program instructions Then implement the situation analysis method based on the locust-optimized LSSVM-ARIMA model described in Example 1.
PUM
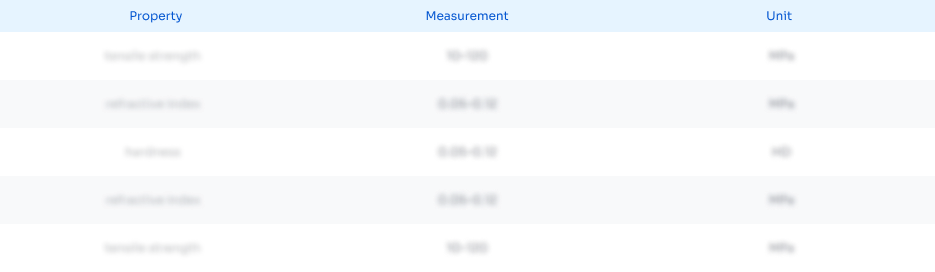
Abstract
Description
Claims
Application Information
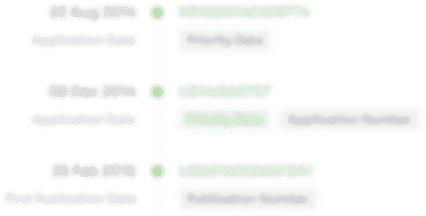
- R&D Engineer
- R&D Manager
- IP Professional
- Industry Leading Data Capabilities
- Powerful AI technology
- Patent DNA Extraction
Browse by: Latest US Patents, China's latest patents, Technical Efficacy Thesaurus, Application Domain, Technology Topic, Popular Technical Reports.
© 2024 PatSnap. All rights reserved.Legal|Privacy policy|Modern Slavery Act Transparency Statement|Sitemap|About US| Contact US: help@patsnap.com