Keyword joint type generation method and system based on semantics and knowledge graph
A knowledge graph and keyword technology, which is applied in the field of combined keyword generation method and system based on semantics and knowledge graph, can solve the problem of inability to handle keyword extraction of headline party articles, difficulty in controlling cost and labeling time, accuracy/coverage Degree/diversity loss etc.
- Summary
- Abstract
- Description
- Claims
- Application Information
AI Technical Summary
Problems solved by technology
Method used
Image
Examples
Embodiment 1
[0127] Such as Figure 1-7 As shown, a joint keyword generation method based on semantic and knowledge graphs, including preparation phase, training phase 1, training phase 2 and inference phase;
[0128] The steps included in the preparatory phase are:
[0129] S11: According to the characteristics of the applicable field, artificially construct the corresponding knowledge map M (such as tree, undirected graph, directed graph) structure to represent the structural relationship of the required keywords (such as inclusion, causality);
[0130] S12: Prepare domain-related unlabeled Chinese corpus of more than 1 million sentences, which are required to contain all the words in the label map M, and the number of occurrences of each word is not less than 50;
[0131] S13: Install sentence vector toolkit sent2vec;
[0132] The steps included in the training phase 1 are:
[0133] S21: clean the prepared corpus, remove special characters, programming language (such as html statemen...
Embodiment 2
[0188] Such as Figure 1-7 As shown, a keyword joint generation system based on semantic and knowledge graph, including preparation unit, training unit 1, training unit 2 and inference unit;
[0189] The preparation unit is used to install the sentence vector toolkit, and the steps included are:
[0190] According to the characteristics of the applicable field, artificially construct the corresponding knowledge map M (such as tree, undirected graph, directed graph) structure to represent the structural relationship of the required keywords (such as inclusion, causality);
[0191] Prepare more than 1 million sentences of unlabeled Chinese corpus related to the field, requiring all the words in the label map M, and the number of occurrences of each word is not less than 50;
[0192] Install the sentence vector toolkit sent2vec;
[0193] The training unit 1 is used to train the sent2vec model, and the steps included are:
[0194] Clean the prepared corpus, remove special chara...
PUM
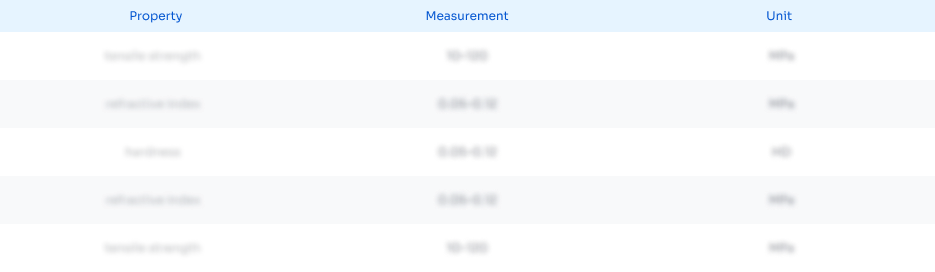
Abstract
Description
Claims
Application Information
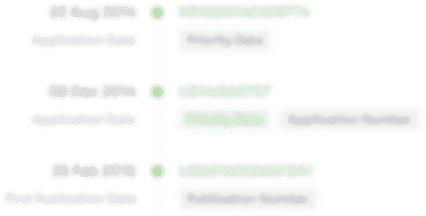
- R&D Engineer
- R&D Manager
- IP Professional
- Industry Leading Data Capabilities
- Powerful AI technology
- Patent DNA Extraction
Browse by: Latest US Patents, China's latest patents, Technical Efficacy Thesaurus, Application Domain, Technology Topic, Popular Technical Reports.
© 2024 PatSnap. All rights reserved.Legal|Privacy policy|Modern Slavery Act Transparency Statement|Sitemap|About US| Contact US: help@patsnap.com