Lithium ion battery remaining service life prediction method based on long and short term memory LSTM and particle filter PF
A lithium-ion battery, life prediction technology, applied in neural learning methods, measuring electricity, measuring devices, etc., can solve problems such as low prediction accuracy and inability to update status
- Summary
- Abstract
- Description
- Claims
- Application Information
AI Technical Summary
Problems solved by technology
Method used
Image
Examples
Embodiment Construction
[0057] Taking the operation data of No. B0005 lithium-ion battery in the NASA public data set shown in Table 1 as an example, the technical solution in the embodiment of the present invention is clearly and completely described in combination with the drawings in the embodiment of the present invention.
[0058] Table 1 B0005 lithium-ion battery
[0059] Constant current charging current / A Charging cut-off voltage / V Discharge current / A Discharge cut-off voltage / V Rated capacity / Ah 1.5 4.2 2.0 2.7 2.0
[0060] Embodiments of the present invention are described in detail below, examples of which are shown in the drawings, wherein the same or similar reference numerals designate the same or similar elements or elements having the same or similar functions throughout. The embodiments described below by referring to the figures are exemplary only for explaining the present invention and should not be construed as limiting the present invention.
[006...
PUM
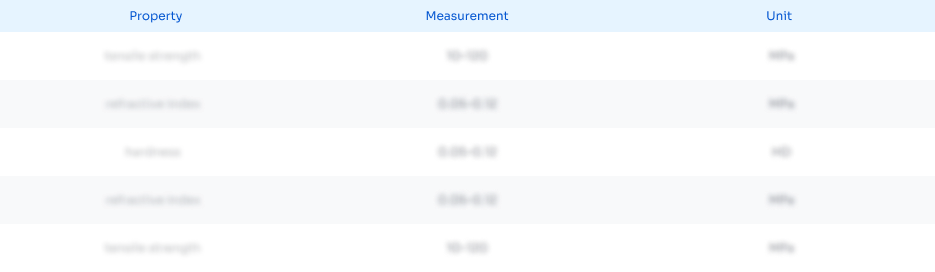
Abstract
Description
Claims
Application Information
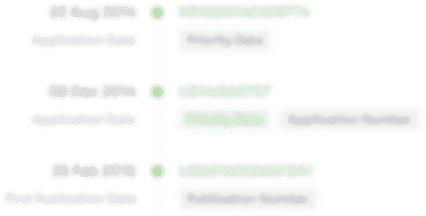
- R&D
- Intellectual Property
- Life Sciences
- Materials
- Tech Scout
- Unparalleled Data Quality
- Higher Quality Content
- 60% Fewer Hallucinations
Browse by: Latest US Patents, China's latest patents, Technical Efficacy Thesaurus, Application Domain, Technology Topic, Popular Technical Reports.
© 2025 PatSnap. All rights reserved.Legal|Privacy policy|Modern Slavery Act Transparency Statement|Sitemap|About US| Contact US: help@patsnap.com