New product demand prediction method
A technology for demand forecasting and new products, applied in forecasting, instruments, computing models, etc., can solve problems such as heterogeneous product differentiation, demand evolution, clustering and forecasting model mismatch, and achieve high accuracy.
- Summary
- Abstract
- Description
- Claims
- Application Information
AI Technical Summary
Problems solved by technology
Method used
Image
Examples
Embodiment 1
[0046] There are 10 new fruit products that are expected to go on sale on April 1, 2019, and the demand for April 1-7, 2019 needs to be forecasted. There are 100 products with historical sales records, then extract product attribute features for these 100 products
[0047] A i =(PL i ,BL i ,C i ,FP i ,FMCG i ,PT i ,PPi ), i∈[1,100]
[0048] where PL i ,BL i ,C i ,FP i ,FMCG i ,PT i ,PP i Indicates the price level, brand level, category, functional parameters, fast-moving consumer goods, packaging type, and origin of product i.
[0049] Extract the feature and demand matrix used for product i model training, assuming product i has t i Duration History
[0050]
[0051] where S j ,j∈[1,t i ] represents the historical demand of product i, element f j,* Represents the eigenvectors corresponding to each historical demand, where the demand is represented by sales.
[0052] Similarly, to extract the attribute features of new products
[0053] NA k =(PL k ,BL...
Embodiment 2
[0077] 5 new women's wear products are expected to go on sale on May 6, 2019, and the demand for May 6-12, 2019 needs to be forecasted. In this case, the demand is calculated by (sales and product launch time) / store sales time. There are currently 200 products with historical sales records, and the product attribute features are extracted from these 200 products
[0078] A i =(PL i ,BL i ,C i ,FP i ,FMCG i ,PT i ,PP i ,ST i , NS i ,NC i ), i∈[1,200]
[0079] A i The 10 fields inside represent the price level, brand level, category, functional parameters, fast moving consumer goods or not, packaging type, origin, style, size quantity, and color quantity of product i respectively.
[0080] Extract the feature and demand matrix used for product i model training, assuming product i has t i Duration History
[0081]
[0082] where S j ,j∈[1,t i ] represents the historical demand of product i, element f j,* Represents the eigenvector corresponding to each histor...
Embodiment 3
[0108] There are 3 new mobile phones that are expected to go on sale on April 15, 2019, and the demand for April 15-21, 2019 needs to be forecasted. In this case, actual sales are used to represent demand. There are 120 products with historical sales records, then extract product attribute features for these 120 products
[0109] A i =(PL i ,BL i ,C i ,FP i ,ZP i ,ST i ,SZ i ,NC i ,PZL i ), i∈[1,120]
[0110] A i The 10 fields inside represent the price level, brand level, category, function parameters, main screen size, style, size, color quantity, and configuration level of the same price of product i respectively.
[0111] Extract the features (all continuous values) and demand matrix used for product i model training, assuming that product i has t i Duration History
[0112]
[0113] where S j ,j∈[1,t i ] represents the historical demand of product i, element f j,* Represents the eigenvector corresponding to each historical demand.
[0114] Similarly, ...
PUM
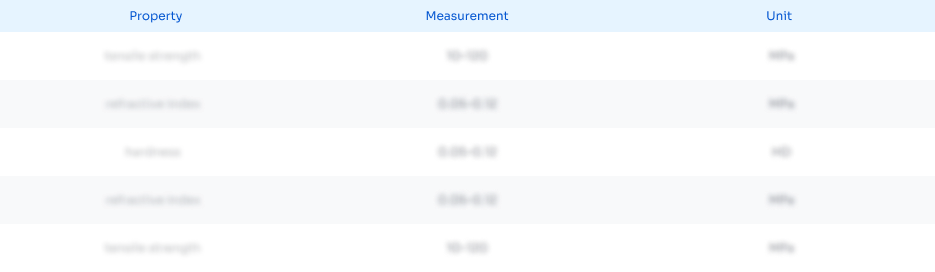
Abstract
Description
Claims
Application Information
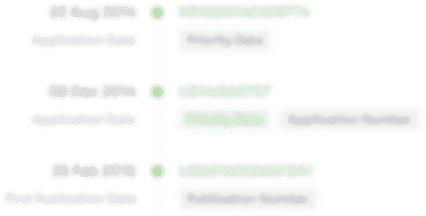
- R&D Engineer
- R&D Manager
- IP Professional
- Industry Leading Data Capabilities
- Powerful AI technology
- Patent DNA Extraction
Browse by: Latest US Patents, China's latest patents, Technical Efficacy Thesaurus, Application Domain, Technology Topic, Popular Technical Reports.
© 2024 PatSnap. All rights reserved.Legal|Privacy policy|Modern Slavery Act Transparency Statement|Sitemap|About US| Contact US: help@patsnap.com