Antenna adjustment method based on reinforcement learning
A technology of reinforcement learning and adjustment methods, applied in wireless communication, radio transmission system, network planning, etc., can solve the problem of sharp increase in air interface utilization efficiency, complexity of network evaluation standards and dynamic adjustment methods of antenna parameters, 3DMIMO and MassiveMIMO networks Problems such as performance evaluation and antenna coverage difficulties, to achieve the effect of solving weight calculation problems, improving network experience, and increasing adjustment speed
- Summary
- Abstract
- Description
- Claims
- Application Information
AI Technical Summary
Problems solved by technology
Method used
Image
Examples
Embodiment Construction
[0014] The present invention will be described in further detail below in conjunction with the accompanying drawings.
[0015] An embodiment of the present invention is an antenna adjustment method based on reinforcement learning, the flow chart is as follows figure 1 As shown, the method includes the following steps:
[0016] S101. Obtain the MDT data reported by the user, and perform grid formation on the user cell;
[0017] S102. Adjust the antenna so that the antenna azimuth beam is aligned with the user clustering direction;
[0018] S103. Calculate the signal coverage parameters of the primary cell based on the gridded MDT data, and determine whether the antenna needs to be adjusted according to the signal coverage parameters of the primary cell; if adjustment is required, go to the next step;
[0019] S104. On the basis of determining the optimization target for antenna adjustment, construct a state set and an action set respectively composed of performance parameters...
PUM
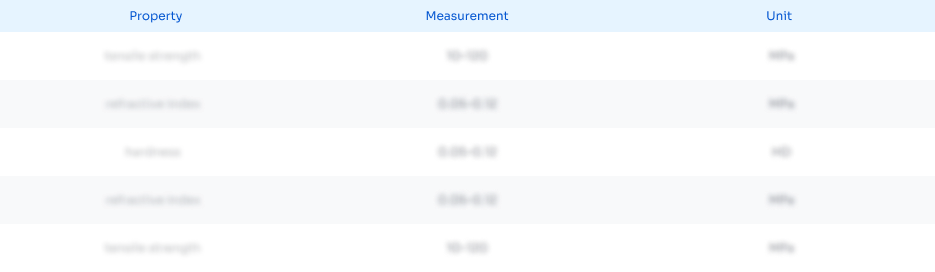
Abstract
Description
Claims
Application Information
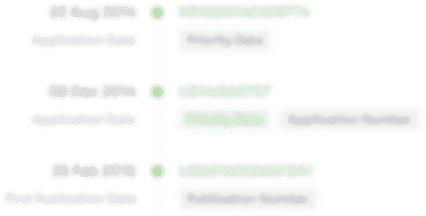
- R&D Engineer
- R&D Manager
- IP Professional
- Industry Leading Data Capabilities
- Powerful AI technology
- Patent DNA Extraction
Browse by: Latest US Patents, China's latest patents, Technical Efficacy Thesaurus, Application Domain, Technology Topic.
© 2024 PatSnap. All rights reserved.Legal|Privacy policy|Modern Slavery Act Transparency Statement|Sitemap