Social network node classification method based on dynamic graph
A social network and node classification technology, applied in the field of data mining, can solve the problem of inability to effectively mine the dependencies between nodes affecting each other at different times, and achieve the effect of improving accuracy
- Summary
- Abstract
- Description
- Claims
- Application Information
AI Technical Summary
Problems solved by technology
Method used
Image
Examples
Embodiment 1
[0048] Social network node classification method based on dynamic graph, such as figure 1 As shown, including the following steps:
[0049] S1. Obtain a tagged social network data set;
[0050] Use social network platforms such as Weibo and Alibaba Cloud Data Platform to collect user's original data, and remove the redundant information, and retain and extract useful information such as user characteristics, behaviors, attributes, tags, etc., to obtain social networks data set:
[0051] X=[x 1 ,x 2 ,x 3 ,...,x m ]
[0052] Where m is the number of sample data contained in the social network data set; let the sample data at time t x t ∈R n , Which contains n variables, and each sample data x t Corresponds to a label variable y t , Where y t ∈L, L represents a collection of label categories.
[0053] S2. Divide the social network data set into T time steps according to time, and construct the adjacency matrix and feature matrix of each time step according to the node attributes and node...
Embodiment 2
[0080] In order to verify the performance of the social network node classification method based on dynamic graphs proposed in Embodiment 1, the following experiments were carried out in this embodiment:
[0081] Use Weibo e-commerce data and Alibaba Cloud platform data as the test data set, where Weibo e-commerce data is homogeneous graph data, and Alibaba Cloud is heterogeneous graph data. In this embodiment, the prediction results of each social network node classification method are compared with the true labels of the test data set, and the classification accuracy rate is calculated. The value range is [0,1]. The higher the value, the better the classification effect. it is good.
[0082] 1. Comparing the social network node classification method of embodiment 1 with the static graph model NRI, GCN, GAT respectively, and the experimental results are as follows: figure 2 Shown. (MyModel in the figure indicates that the method of embodiment 1 is used), it can be seen that the ...
PUM
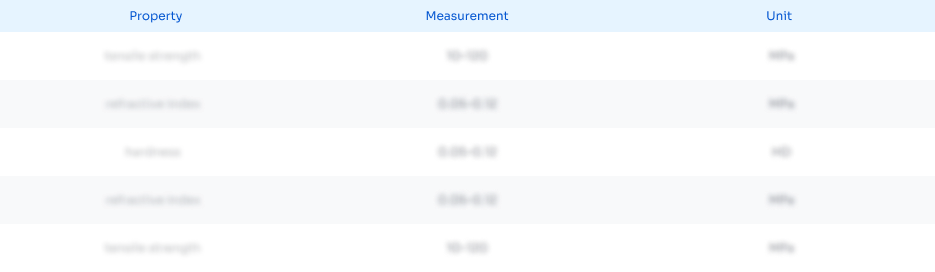
Abstract
Description
Claims
Application Information
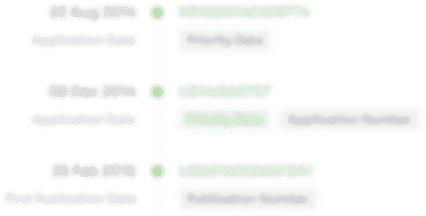
- R&D Engineer
- R&D Manager
- IP Professional
- Industry Leading Data Capabilities
- Powerful AI technology
- Patent DNA Extraction
Browse by: Latest US Patents, China's latest patents, Technical Efficacy Thesaurus, Application Domain, Technology Topic, Popular Technical Reports.
© 2024 PatSnap. All rights reserved.Legal|Privacy policy|Modern Slavery Act Transparency Statement|Sitemap|About US| Contact US: help@patsnap.com