PHD multi-target tracking method based on variational Bayesian T distributed Kalman filtering
A variational Bayesian and Kalman filtering technology, applied in the field of multi-target tracking and robust PHD tracking, it can solve the problem that it is difficult to effectively handle measurement outliers, the robustness of the filtering algorithm is not strong, and the measurement abnormality cannot be optimized. questions of value
- Summary
- Abstract
- Description
- Claims
- Application Information
AI Technical Summary
Problems solved by technology
Method used
Image
Examples
Embodiment Construction
[0066] The technical solution of the present invention will be further described in detail below in conjunction with the accompanying drawings.
[0067] The basic theory involved in the present invention is introduced as follows:
[0068] 1. Variational Bayesian inference techniques
[0069] Assuming that the measurement is Z and the target state is X, in the recursive process, if you want to use the function Ψ(Z) to approximate the posterior probability density g(Z|X), you can iteratively approximate it by reducing the KL divergence, that is
[0070]
[0071] make
[0072] L(Ψ)=∫Ψ(Z)logg(Z,X)dZ-∫Ψ(Z)logΨ(Z)dZ
[0073] Then Ψ(Z) can be expressed as logg(X)=KL(Ψ||g)+L(Ψ)
[0074] So the problem is transformed into how to minimize KL(Ψ||g). Obviously, if you want to minimize KL(Ψ||g), you only need to maximize L(Ψ). Assuming that the hidden variables are independent of each other, then L(Ψ) can be expressed as
[0075]
[0076] Let -KL(Ψ(z j )||Ψ * (z j ))=0, then...
PUM
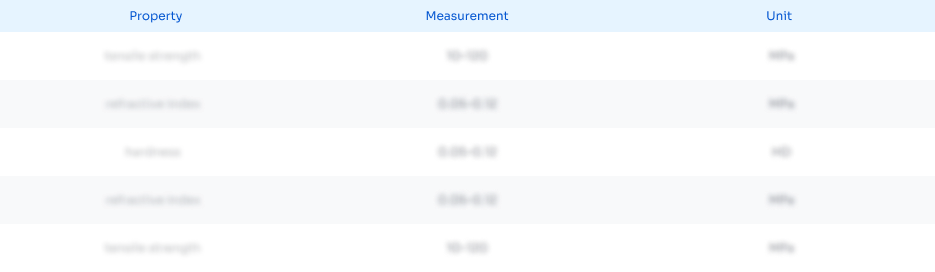
Abstract
Description
Claims
Application Information
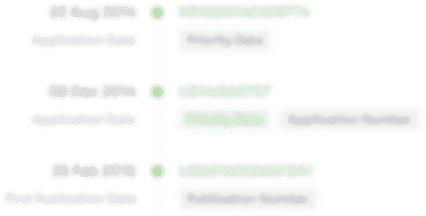
- R&D Engineer
- R&D Manager
- IP Professional
- Industry Leading Data Capabilities
- Powerful AI technology
- Patent DNA Extraction
Browse by: Latest US Patents, China's latest patents, Technical Efficacy Thesaurus, Application Domain, Technology Topic, Popular Technical Reports.
© 2024 PatSnap. All rights reserved.Legal|Privacy policy|Modern Slavery Act Transparency Statement|Sitemap|About US| Contact US: help@patsnap.com