Hyperspectral remote sensing image classification method based on dense residual three-dimensional convolutional neural network
A hyperspectral remote sensing and neural network technology, applied in the field of remote sensing mapping and information engineering, can solve the problems of unsatisfactory classification accuracy and complex information, and achieve the effect of reducing training time, increasing the overall number, and enhancing feature transfer.
- Summary
- Abstract
- Description
- Claims
- Application Information
AI Technical Summary
Problems solved by technology
Method used
Image
Examples
Embodiment Construction
[0044] The technical solutions of the present invention will be further described below in conjunction with the accompanying drawings and embodiments.
[0045] A hyperspectral remote sensing image classification method based on a dense residual three-dimensional convolutional neural network (DR-3D-CNN) according to the present invention, the schematic diagram of the network structure is as follows figure 1 shown, including the following steps:
[0046] Step 1, using the original sample of the hyperspectral remote sensing image to construct a virtual sample, mixing the original sample and the virtual sample to form a training sample, the training sample is a three-dimensional data cube; the details are as follows:
[0047] Using the original sample data of the hyperspectral remote sensing image through simulated imaging, random factors and random noise are added to the original sample to form a virtual sample. The virtual sample is a pseudo sample transformed from the original ...
PUM
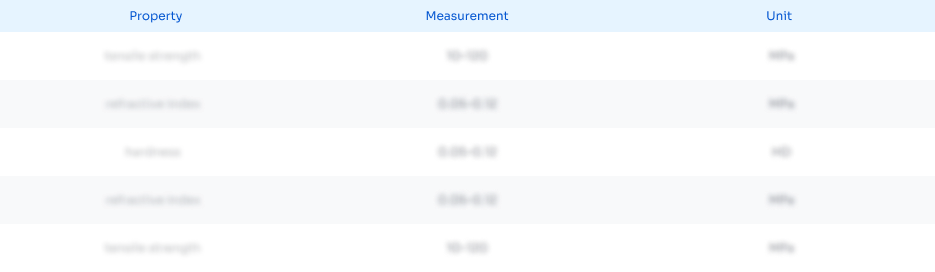
Abstract
Description
Claims
Application Information
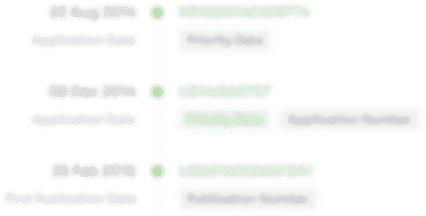
- R&D
- Intellectual Property
- Life Sciences
- Materials
- Tech Scout
- Unparalleled Data Quality
- Higher Quality Content
- 60% Fewer Hallucinations
Browse by: Latest US Patents, China's latest patents, Technical Efficacy Thesaurus, Application Domain, Technology Topic, Popular Technical Reports.
© 2025 PatSnap. All rights reserved.Legal|Privacy policy|Modern Slavery Act Transparency Statement|Sitemap|About US| Contact US: help@patsnap.com