Cell nucleus center point detection method based on multi-task convolutional neural network
A convolutional neural network and detection method technology, applied in the field of cell nucleus center point detection based on multi-task convolutional neural network, can solve the problems of slow convergence, false negatives, false positives, etc., to improve detection performance and increase data annotation effect of difficulty
- Summary
- Abstract
- Description
- Claims
- Application Information
AI Technical Summary
Problems solved by technology
Method used
Image
Examples
Embodiment Construction
[0052] In order to make the object, technical solution and advantages of the present invention clearer, the present invention will be further described in detail below in conjunction with the accompanying drawings.
[0053] Briefly, the invention includes data preparation, model structure design and model post-processing.
[0054] Data preparation: When labeling data, directly mark the center point of the cell nucleus. The two types of masks during training can be generated directly according to the coordinates of the point. The single-type Gaussian kernel is a two-dimensional Gaussian distribution in which the center point coincides with the marked center point. Array, the two types of equivalent cores are two-dimensional arrays of equivalent circular structure elements whose center point coincides with the label data.
[0055] Model structure design: This solution uses a fully convolutional network structure, including but not limited to the classic FCN network structure. Fi...
PUM
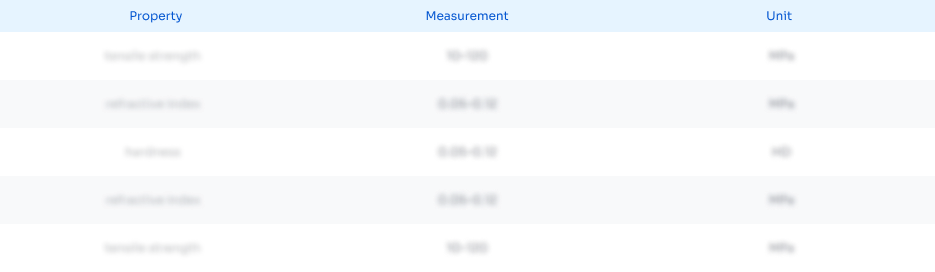
Abstract
Description
Claims
Application Information
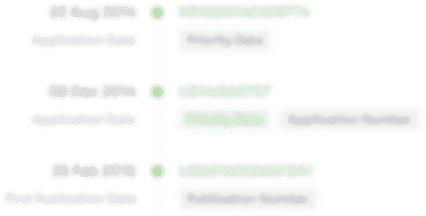
- R&D Engineer
- R&D Manager
- IP Professional
- Industry Leading Data Capabilities
- Powerful AI technology
- Patent DNA Extraction
Browse by: Latest US Patents, China's latest patents, Technical Efficacy Thesaurus, Application Domain, Technology Topic, Popular Technical Reports.
© 2024 PatSnap. All rights reserved.Legal|Privacy policy|Modern Slavery Act Transparency Statement|Sitemap|About US| Contact US: help@patsnap.com