Semi-supervised Mongolian-Chinese neural machine translation method based on collaborative training
A collaborative training and machine translation technology, applied in the field of artificial intelligence, can solve the problems of unavailable translation results, lack of high quality, large scale, wide coverage, poor performance of neural machine translation, etc., to alleviate scarcity and improve accuracy Effect
- Summary
- Abstract
- Description
- Claims
- Application Information
AI Technical Summary
Problems solved by technology
Method used
Image
Examples
Embodiment Construction
[0050] The invention provides a semi-supervised Mongolian-Chinese neural machine translation method based on collaborative training. The collaborative training method is a method of gradually expanding the original corpus by rationally using the existing monolingual corpus under the condition that the original parallel corpus is scarce. method. The present invention first uses Mongolian-Chinese (mo-ch), English-Chinese (en-ch) and Korean-Chinese (ko-ch) parallel corpora, and constructs three initial translation models based on semi-supervised classification and generative adversarial networks: Mongolian-Chinese translation model M -mo-ch, English-Chinese translation model M-en-ch and Korean-Chinese translation model M-ko-ch, and use these three translation models to perform multi-source mutual parallel corpus Mongolian-English-Korean (mo-en-ko) to The target end is the mark of Chinese (ch), and the best quality marked corpus is selected by using the language model LM-ch traine...
PUM
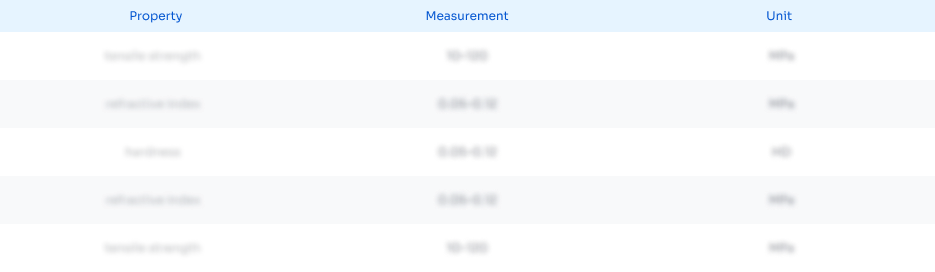
Abstract
Description
Claims
Application Information
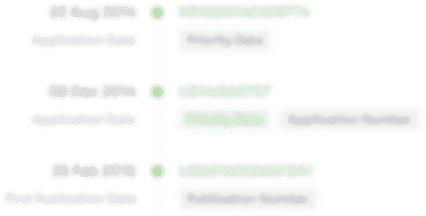
- R&D Engineer
- R&D Manager
- IP Professional
- Industry Leading Data Capabilities
- Powerful AI technology
- Patent DNA Extraction
Browse by: Latest US Patents, China's latest patents, Technical Efficacy Thesaurus, Application Domain, Technology Topic, Popular Technical Reports.
© 2024 PatSnap. All rights reserved.Legal|Privacy policy|Modern Slavery Act Transparency Statement|Sitemap|About US| Contact US: help@patsnap.com