Big data filtering method based on multi-core fusion
A technology of multi-core fusion and big data, applied in the direction of impedance network, adaptive network, electrical components, etc., can solve problems such as performance limitations, and achieve good convergence speed and excellent estimation performance
- Summary
- Abstract
- Description
- Claims
- Application Information
AI Technical Summary
Problems solved by technology
Method used
Image
Examples
Embodiment Construction
[0050] The technical solutions of the present invention will be further described below through the embodiments and accompanying drawings.
[0051] see figure 2 with image 3 , a big data filtering method based on multi-core fusion, the steps are as follows:
[0052] Step 1: For each filter initialization, set the parameters of each kernel adaptive filter separately, including kernel width σ, regularization factor γ, and initial fusion coefficient α.
[0053] Step 2: Update the parameter data of each filter according to the single-core adaptive filtering algorithm, and calculate the weight coefficient a at the i-th moment i .
[0054] Step 3: Update the fusion coefficient while training the filter weight coefficient. The fusion coefficient of the m-th filter is the posterior probability of the kernel width when the weight coefficient is calculated as valid. The fusion coefficient must meet the threshold limit.
[0055] Step 4: Perform weighted fusion of multiple independe...
PUM
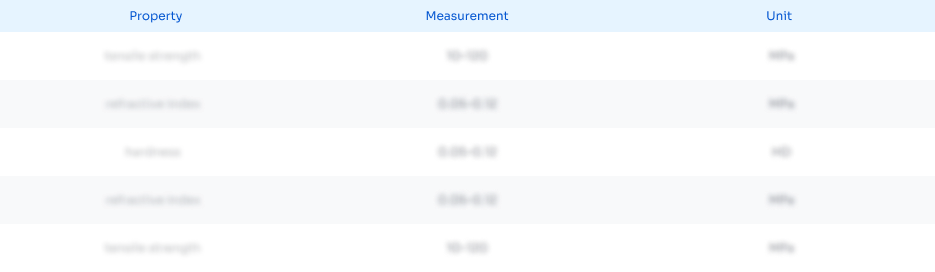
Abstract
Description
Claims
Application Information
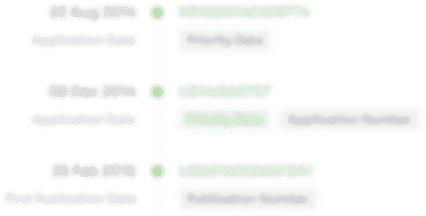
- R&D Engineer
- R&D Manager
- IP Professional
- Industry Leading Data Capabilities
- Powerful AI technology
- Patent DNA Extraction
Browse by: Latest US Patents, China's latest patents, Technical Efficacy Thesaurus, Application Domain, Technology Topic, Popular Technical Reports.
© 2024 PatSnap. All rights reserved.Legal|Privacy policy|Modern Slavery Act Transparency Statement|Sitemap|About US| Contact US: help@patsnap.com