Real-time semantic segmentation method with low calculation amount and high feature fusion
A feature fusion and semantic segmentation technology, applied in the field of computer vision, can solve problems such as huge computational load, and achieve the effect of improving feature utilization and satisfying computational accuracy.
- Summary
- Abstract
- Description
- Claims
- Application Information
AI Technical Summary
Problems solved by technology
Method used
Image
Examples
specific Embodiment approach
[0042] Step 1: Construct a semantic segmentation network based on an "encoder-decoder" multi-branch structure. figure 1 Given the network structure diagram of the semantic segmentation constructed in the present invention, in the "encoder" part, the network consists of three branches, and in each branch, as the network deepens, deeper features are extracted, but the features The size of the graph is continuously reduced; in the "decoder" part, the network combines the features of different stages extracted by the "encoder" in a splicing way and performs upsampling of corresponding multiples to restore the size of the feature map, and finally get the same as the original Semantic segmentation result map with same image size.
[0043] Step 2: Use images of different resolutions as the input of each branch of the multi-branch in the "encoder". figure 1 The given semantic segmentation network structure diagram of the present invention shows that the original size image, 2x downsa...
PUM
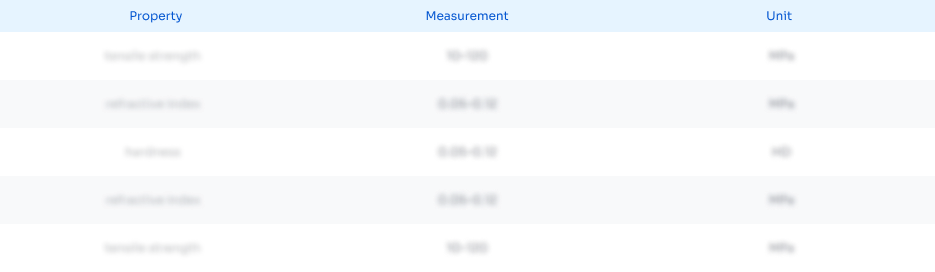
Abstract
Description
Claims
Application Information
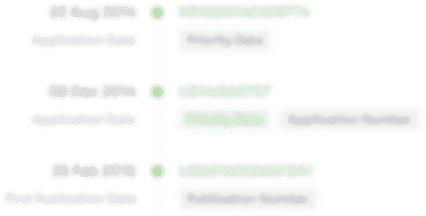
- R&D Engineer
- R&D Manager
- IP Professional
- Industry Leading Data Capabilities
- Powerful AI technology
- Patent DNA Extraction
Browse by: Latest US Patents, China's latest patents, Technical Efficacy Thesaurus, Application Domain, Technology Topic, Popular Technical Reports.
© 2024 PatSnap. All rights reserved.Legal|Privacy policy|Modern Slavery Act Transparency Statement|Sitemap|About US| Contact US: help@patsnap.com