Hesitant fuzzy and dynamic deep reinforcement learning combined unmanned aerial vehicle maneuvering decision-making method
A technology of reinforcement learning and decision-making methods, applied in multi-objective optimization, design optimization/simulation, CAD numerical modeling, etc., can solve problems such as fuzzy and inaccurate optimization target parameters, and achieve reasonable decision-making results
- Summary
- Abstract
- Description
- Claims
- Application Information
AI Technical Summary
Problems solved by technology
Method used
Image
Examples
Embodiment Construction
[0025] The technical solution of the present invention is described in detail in combination with the accompanying drawings.
[0026] The UAV maneuver decision-making method combining hesitant fuzzy and dynamic deep reinforcement learning of the present invention specifically includes the following steps:
[0027] Step 1, establish the UAV air combat movement model, according to the missile attack parameters ξ of both the enemy and the enemy i , ξ T and the energy parameter difference ΔW based on the situation, establish a decision-making model based on the weighted optimization objective, specifically:
[0028] (1.1) The UCAV is regarded as a particle, regardless of the specific rigid body motion and flight control algorithm, the three-degree-of-freedom particle model is used to describe its motion state, and its motion model is:
[0029]
[0030] In the formula, x, y, z represent the position of the aircraft in the inertial coordinate system, v is the flight speed of th...
PUM
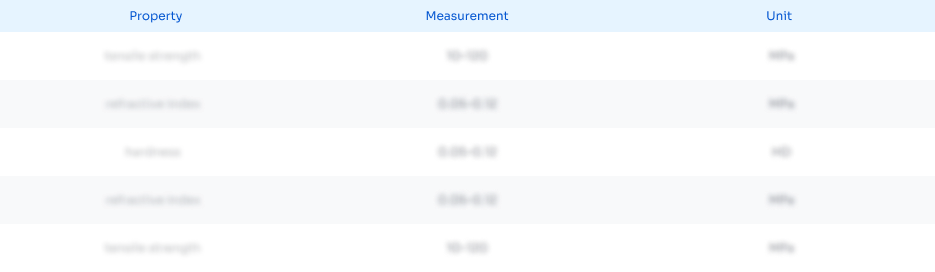
Abstract
Description
Claims
Application Information
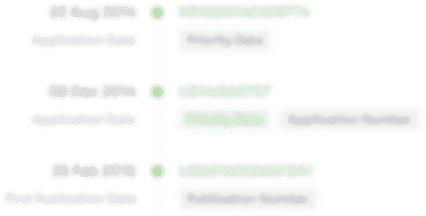
- R&D Engineer
- R&D Manager
- IP Professional
- Industry Leading Data Capabilities
- Powerful AI technology
- Patent DNA Extraction
Browse by: Latest US Patents, China's latest patents, Technical Efficacy Thesaurus, Application Domain, Technology Topic, Popular Technical Reports.
© 2024 PatSnap. All rights reserved.Legal|Privacy policy|Modern Slavery Act Transparency Statement|Sitemap|About US| Contact US: help@patsnap.com