Traffic sign and signal lamp detection and identification optimization algorithm
A traffic sign, optimization algorithm technology, applied in character and pattern recognition, calculation, computer parts and other directions, can solve the problems of difficult to meet real-time requirements, the amount of calculation cannot be reduced, and the calculation cost is high, to improve the classification accuracy, The effect of reducing feature redundancy and fast forward inference
- Summary
- Abstract
- Description
- Claims
- Application Information
AI Technical Summary
Problems solved by technology
Method used
Image
Examples
Embodiment 1
[0069] Use the test set Tsinghua-Tencent 100K of the author of the baseline algorithm to do multiple comparison experiments. The test set has a total of 1,500 images and a total of 3,120 traffic signs and traffic lights. Using the benchmark used by the authors for areas smaller than 32 2 (small), with an area of 32 2 to 96 2 (middle), the area is greater than 96 2 The (big) detection results are counted separately, the intersection-over-union ratio (IoU) threshold is set to 0.5, and its precision rate, recall rate, and mAP are calculated respectively. In addition, in order to compare and study the calculation amount and memory consumption of the present invention, the baseline algorithm Statistics are carried out with the average forward inference speed, memory usage and model size of the present invention.
[0070] Table 1 Performance reference table for various aspects of comparative experiments
[0071]
[0072]
[0073] Compared with the baseline method: the pr...
PUM
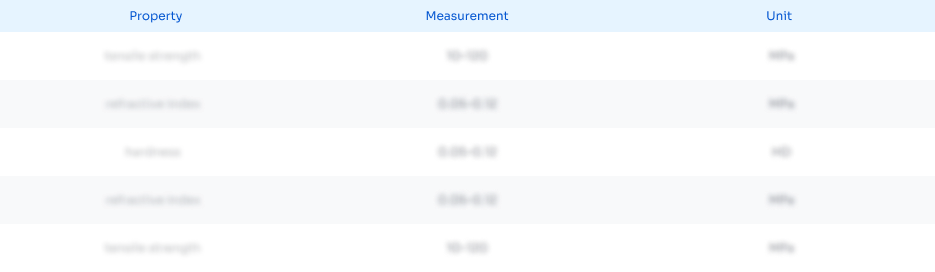
Abstract
Description
Claims
Application Information
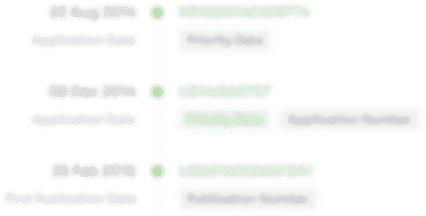
- R&D Engineer
- R&D Manager
- IP Professional
- Industry Leading Data Capabilities
- Powerful AI technology
- Patent DNA Extraction
Browse by: Latest US Patents, China's latest patents, Technical Efficacy Thesaurus, Application Domain, Technology Topic, Popular Technical Reports.
© 2024 PatSnap. All rights reserved.Legal|Privacy policy|Modern Slavery Act Transparency Statement|Sitemap|About US| Contact US: help@patsnap.com