Deep learning-based high beam identification method
A technology of deep learning and recognition method, applied in the field of high beams, can solve problems such as dazzling phenomenon, and achieve the effect of avoiding stray light interference, reducing feature redundancy, and reducing complexity
- Summary
- Abstract
- Description
- Claims
- Application Information
AI Technical Summary
Problems solved by technology
Method used
Image
Examples
Embodiment 1
[0029] This embodiment discloses as figure 1 A high beam recognition method based on deep learning is shown, comprising the following steps:
[0030] S1 Set the camera in the area to be tested, and adjust its parameters such as height, pitch angle and camera exposure to meet the forensic distance;
[0031] S2 sets the appropriate exposure, highlights the halo area of the car light, reduces the adhesion of the high beam in the image, and at the same time ensures the clarity of the vehicle license plate under the fill light;
[0032] S3 extracts features such as center brightness, edge symmetry, and linear gradient that characterize the image information of the light spot and halo, and judges the category of the car light;
[0033] S4 extracts and classifies car light categories based on multi-objective convolutional neural networks, and uses deep learning algorithms to achieve multi-task learning;
[0034] S5 uses the improved DBSCAN algorithm combined with the least square...
Embodiment 2
[0042] This embodiment discloses as figure 2 The vehicle high-beam recognition verification setting shown is a typical straight-going vehicle scene, H is the height of the monitoring pole, α is the camera’s overlooking angle, β is the vertical field of view of the lens, and points A, B, and C are the points on the road. mark point. Points C to A are the effective detection range of the high beam. When tracking the high beam vehicle between AC, when the high beam lasts for a long time or the driving distance is long, it is regarded as misusing the high beam.
[0043] In this embodiment, parameters such as camera height H, pitch angle α, and camera exposure are first set to meet the evidence collection distance. Set the appropriate exposure to highlight the halo area of the car light, reduce the adhesion of the high beam in the image, and at the same time ensure the clarity of the vehicle license plate under the fill light. Secondly, the headlight area is preliminarily scre...
Embodiment 3
[0046] In this embodiment, when detecting night lights, extracting the multi-dimensional features of car lights requires separating the spot and halo area in advance. The average initial attenuation brightness of the high-beam car lights in the statistical database is used as the image segmentation threshold, and the narrow and long closed connected domains are filtered out. Preliminary detection The light spot of the car light, the light spot diverges outward in a circular shape to obtain the corresponding halo area. When the traffic flow is dense at night, the halos under the headlights and the left and right halos are easily damaged by ground reflections, body reflections, etc., and the linear structure jumps. Select the region of interest extracted from the upper half fan of the spot centroid as a linear feature, extract stable halo gradient features, and reduce stray light interference.
[0047] In this embodiment, when extracting car lights and visual object detection, t...
PUM
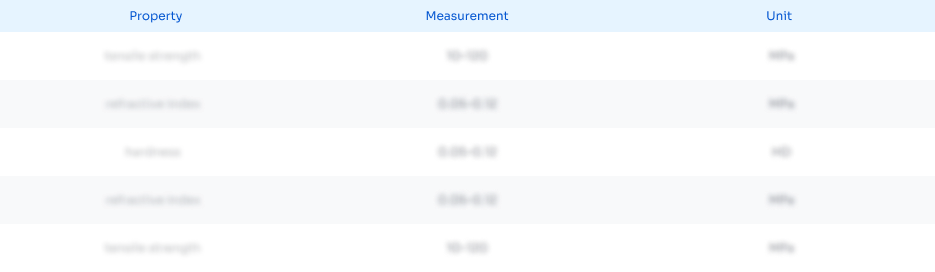
Abstract
Description
Claims
Application Information
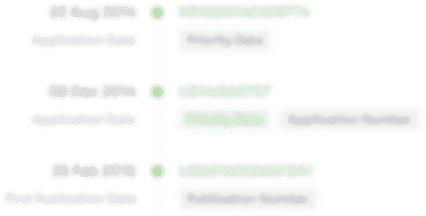
- R&D Engineer
- R&D Manager
- IP Professional
- Industry Leading Data Capabilities
- Powerful AI technology
- Patent DNA Extraction
Browse by: Latest US Patents, China's latest patents, Technical Efficacy Thesaurus, Application Domain, Technology Topic, Popular Technical Reports.
© 2024 PatSnap. All rights reserved.Legal|Privacy policy|Modern Slavery Act Transparency Statement|Sitemap|About US| Contact US: help@patsnap.com