Non-contact automatic mapping method based on deep learning
A non-contact, deep learning technology, applied in the field of deep learning, can solve the problems of time-consuming, unaligned, low quality, etc., to achieve the effect of reducing manual steps and speeding up the texture speed
- Summary
- Abstract
- Description
- Claims
- Application Information
AI Technical Summary
Problems solved by technology
Method used
Image
Examples
Embodiment Construction
[0040] The present invention will be further described below in conjunction with the accompanying drawings and specific embodiments, but the following embodiments in no way limit the present invention.
[0041] The present invention proposes a non-contact automatic mapping method based on deep learning, and the design idea is to use the deep learning method to replace the traditional method of using calibration point pairs to obtain camera parameters. The basic steps of the method implementation are: figure 1 As shown, first prepare the object to be collected, the camera used for collection and the 3D model of the object, and then pass the Zhang Zhengyou calibration method [1] Calibrate the camera. During the calibration process, the color test card is used as the calibration board. After the calibration, the internal parameters of the camera are obtained. However, since the focal length needs to be changed during the process of collecting each image, the focal length paramete...
PUM
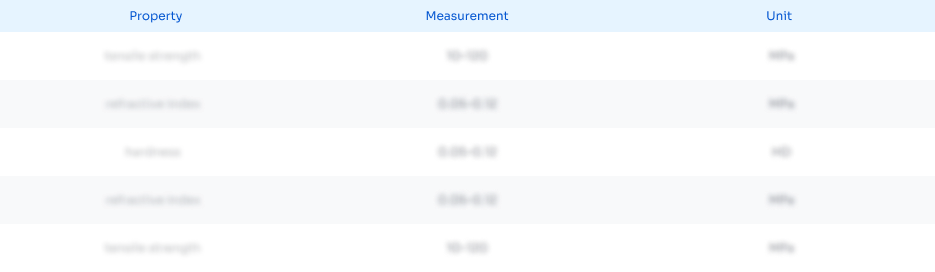
Abstract
Description
Claims
Application Information
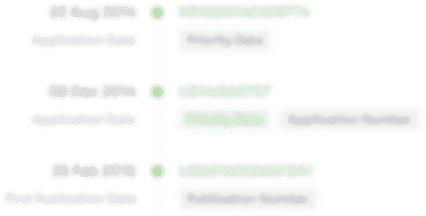
- R&D Engineer
- R&D Manager
- IP Professional
- Industry Leading Data Capabilities
- Powerful AI technology
- Patent DNA Extraction
Browse by: Latest US Patents, China's latest patents, Technical Efficacy Thesaurus, Application Domain, Technology Topic, Popular Technical Reports.
© 2024 PatSnap. All rights reserved.Legal|Privacy policy|Modern Slavery Act Transparency Statement|Sitemap|About US| Contact US: help@patsnap.com