Semi-supervised deep network picture classification method based on swarm intelligence
A deep network and image classification technology, which is applied in still image data clustering/classification, neural learning methods, biological neural network models, etc., can solve problems that cannot be effectively fed back by supervised models
- Summary
- Abstract
- Description
- Claims
- Application Information
AI Technical Summary
Problems solved by technology
Method used
Image
Examples
Embodiment Construction
[0041] The present invention will be further described below in combination with specific embodiments.
[0042] The invention uses a semi-supervised deep neural network classification method and a group optimization particle swarm optimization algorithm to identify and classify digital picture data; overcomes the defects that the full-supervised method requires more marking information and consumes a lot of materials, and also overcomes the general semi-supervised method The method has the defect of low classification accuracy due to no good feedback on unlabeled data; the present invention adopts the particle swarm optimization algorithm in group optimization, uses multiple supervised models to perform different initializations and adds noise, and fully explores the correlation between data The accuracy of the algorithm is improved; the visualization of the intermediate process of the present invention is more helpful to the understanding and analysis of deep learning workers;...
PUM
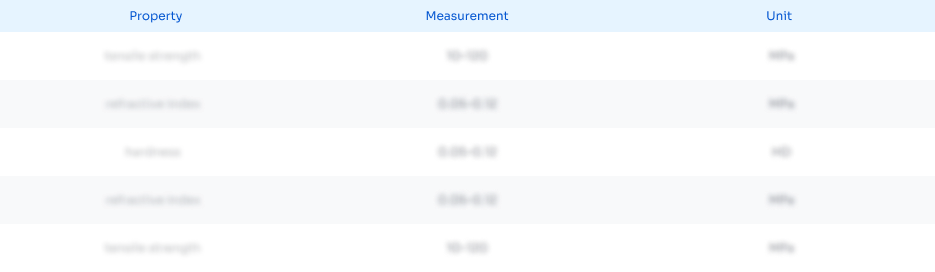
Abstract
Description
Claims
Application Information
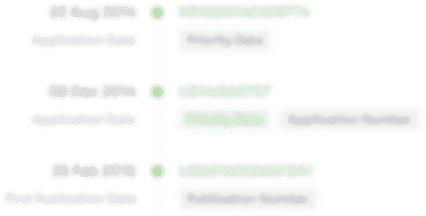
- R&D
- Intellectual Property
- Life Sciences
- Materials
- Tech Scout
- Unparalleled Data Quality
- Higher Quality Content
- 60% Fewer Hallucinations
Browse by: Latest US Patents, China's latest patents, Technical Efficacy Thesaurus, Application Domain, Technology Topic, Popular Technical Reports.
© 2025 PatSnap. All rights reserved.Legal|Privacy policy|Modern Slavery Act Transparency Statement|Sitemap|About US| Contact US: help@patsnap.com