Rolling bearing transfer learning fault diagnosis method based on partial domain adversarial
A rolling bearing and transfer learning technology, applied in machine learning, mechanical bearing testing, computer parts, etc., can solve problems such as no way to train weighted models
- Summary
- Abstract
- Description
- Claims
- Application Information
AI Technical Summary
Problems solved by technology
Method used
Image
Examples
Embodiment Construction
[0072] The present invention will be further described below in conjunction with the accompanying drawings and embodiments.
[0073] Such as figure 1 As shown, a rolling bearing transfer learning fault diagnosis method based on partial domain confrontation includes the following steps:
[0074] (1) Build a rolling bearing fault data sample library under different working conditions, divide the source domain and target domain fault data, and use the source domain samples as training data and the target domain samples as test data.
[0075] The source domain data is The target domain data is where x (i) (i=1,2,...,n s ) is a labeled sample in the source domain, y (i) (i=1,2,...,n s ) is the label of the source domain sample, x (j) (j=1,2,...,n t ) is an unlabeled sample in the target domain; n s and n t are the sample sizes of the source and target domains, respectively. Sample label type in Indicates the number of sample labels in the target domain, Indicates...
PUM
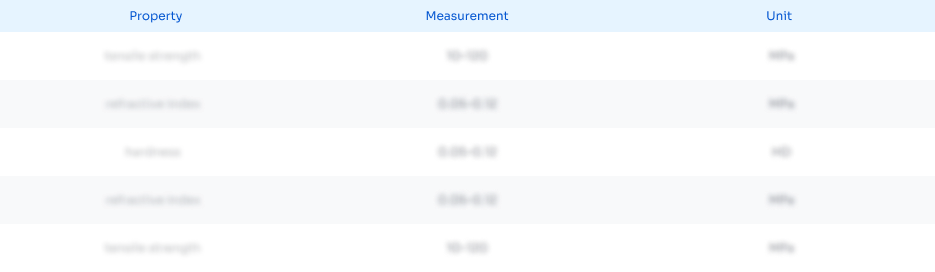
Abstract
Description
Claims
Application Information
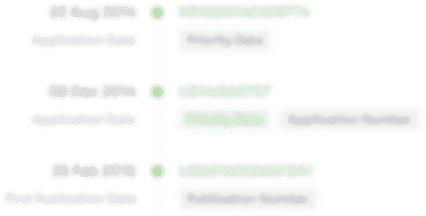
- R&D Engineer
- R&D Manager
- IP Professional
- Industry Leading Data Capabilities
- Powerful AI technology
- Patent DNA Extraction
Browse by: Latest US Patents, China's latest patents, Technical Efficacy Thesaurus, Application Domain, Technology Topic, Popular Technical Reports.
© 2024 PatSnap. All rights reserved.Legal|Privacy policy|Modern Slavery Act Transparency Statement|Sitemap|About US| Contact US: help@patsnap.com