Cloth defect detection method based on deep learning multi-layer feature fusion
A technology of feature fusion and deep learning, applied in neural learning methods, image data processing, image enhancement, etc., can solve problems such as difficulty in meeting industrial requirements, large amount of calculation, and reduced cloth defect detection rate
- Summary
- Abstract
- Description
- Claims
- Application Information
AI Technical Summary
Problems solved by technology
Method used
Image
Examples
Embodiment Construction
[0043] The following will clearly and completely describe the technical solutions in the embodiments of the present invention with reference to the accompanying drawings in the embodiments of the present invention. Obviously, the described embodiments are only some, not all, embodiments of the present invention.
[0044] Such as figure 1 As shown, a cloth defect detection method based on deep learning multi-layer feature fusion includes the following steps:
[0045] S01: Establish a cloth inspection image library and its label library for different defect categories, and import defect category pictures;
[0046] S02: Using data augmentation technology to expand the data in the cloth detection image library;
[0047] S03: Divide the expanded cloth detection image library data into a training set and a test set, and process the data;
[0048] S04: Design a target detection network model for multi-layer feature fusion;
[0049] S05: Use the training set to train the designed n...
PUM
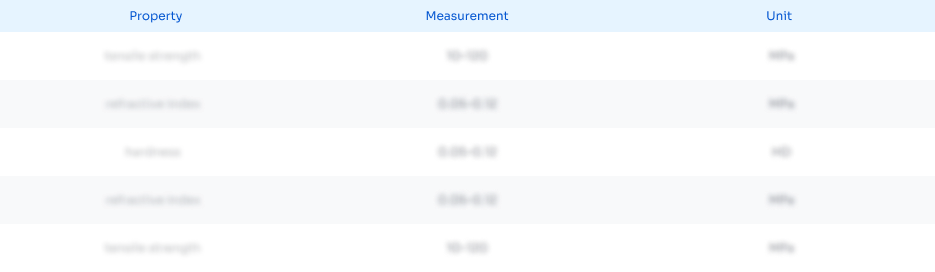
Abstract
Description
Claims
Application Information
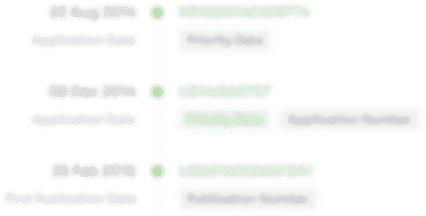
- R&D Engineer
- R&D Manager
- IP Professional
- Industry Leading Data Capabilities
- Powerful AI technology
- Patent DNA Extraction
Browse by: Latest US Patents, China's latest patents, Technical Efficacy Thesaurus, Application Domain, Technology Topic, Popular Technical Reports.
© 2024 PatSnap. All rights reserved.Legal|Privacy policy|Modern Slavery Act Transparency Statement|Sitemap|About US| Contact US: help@patsnap.com