Unified reinforcement learning method based on curiosity driving
A technology of reinforcement learning and curiosity, applied in the field of reinforcement learning, can solve the problems of affecting the efficiency of learning, inaccurate results, and the inability of internal rewards to fully and effectively guide the agent to explore and learn.
- Summary
- Abstract
- Description
- Claims
- Application Information
AI Technical Summary
Problems solved by technology
Method used
Image
Examples
Embodiment
[0083] The implementation method of this embodiment is as described above, and the specific steps will not be described in detail. The following only shows the effect of the case data.
[0084] First use the attention module to obtain a reliable feature representation of the state, and then use state novelty estimation and forward dynamic prediction to estimate the degree of exploration of the state and state-action pairs, that is, the internal reward of the initial estimate. On this basis, the estimated internal rewards are smoothed using multiple samples in the state space, and different types of internal rewards are fused to obtain more accurate and robust internal rewards. Finally, the agent learns the policy using the empirical data generated by interacting with the environment and the estimated internal rewards. The result is asfigure 1 , 2 , 3 shown.
[0085] figure 1 The result of visualizing the features extracted by the attention module of the present invention on...
PUM
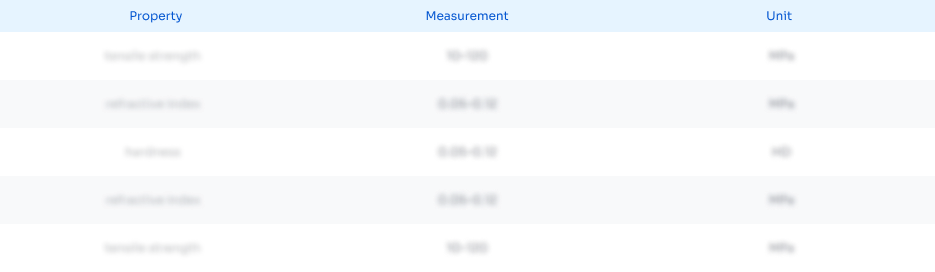
Abstract
Description
Claims
Application Information
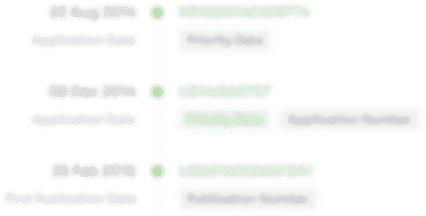
- R&D Engineer
- R&D Manager
- IP Professional
- Industry Leading Data Capabilities
- Powerful AI technology
- Patent DNA Extraction
Browse by: Latest US Patents, China's latest patents, Technical Efficacy Thesaurus, Application Domain, Technology Topic.
© 2024 PatSnap. All rights reserved.Legal|Privacy policy|Modern Slavery Act Transparency Statement|Sitemap