DSM local missing repairing method based on deep learning
A repair method and deep learning technology, applied in image data processing, instruments, biological neural network models, etc., can solve the problems of complex process and low accuracy, and achieve the effect of improving repair accuracy and enhancing extraction ability.
- Summary
- Abstract
- Description
- Claims
- Application Information
AI Technical Summary
Problems solved by technology
Method used
Image
Examples
Embodiment Construction
[0046] The specific embodiments of the present invention will be further described below in conjunction with the accompanying drawings.
[0047] The repair method of the present invention applies the deep learning image repair method to DSM repair. The algorithm can effectively reduce the repair error by combining partial convolution and attention modules on the basis of U-Net, and has better robustness sex. Among them, partial convolution can enhance the ability to extract irregular and missing edge features; the attention module can increase the feature weight adaptive learning mechanism in the two dimensions of channel and space.
[0048] Specifically, the repair model of the present invention uses a partially convolutional U-Net network plus an attention module, such as figure 1 As shown, it includes three modules: feature extraction module, channel fusion module and resolution restoration module. The input DSM first extracts features layer by layer through the feature e...
PUM
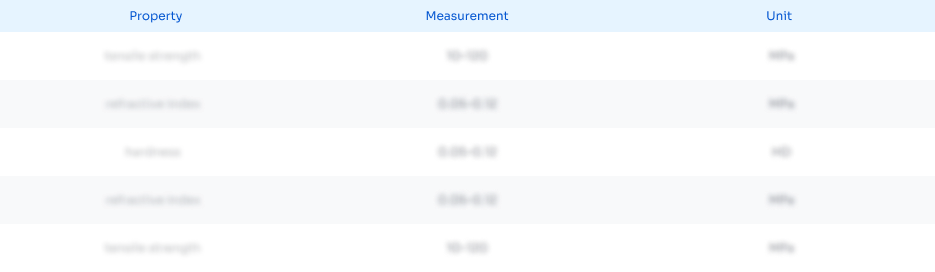
Abstract
Description
Claims
Application Information
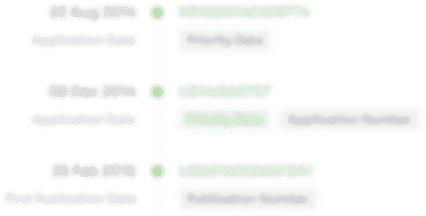
- R&D Engineer
- R&D Manager
- IP Professional
- Industry Leading Data Capabilities
- Powerful AI technology
- Patent DNA Extraction
Browse by: Latest US Patents, China's latest patents, Technical Efficacy Thesaurus, Application Domain, Technology Topic, Popular Technical Reports.
© 2024 PatSnap. All rights reserved.Legal|Privacy policy|Modern Slavery Act Transparency Statement|Sitemap|About US| Contact US: help@patsnap.com