Tunnel identification model and method based on multi-scale edge feature detection
A technology of edge features and recognition models, applied in the field of computer image processing, can solve problems such as unsatisfactory recognition accuracy and insufficient use of multi-scale information
- Summary
- Abstract
- Description
- Claims
- Application Information
AI Technical Summary
Problems solved by technology
Method used
Image
Examples
Embodiment
[0043] Such as Figure 4 As shown, in this embodiment, the specific implementation steps of a tunnel identification method based on multi-scale edge feature detection are as follows:
[0044] S1. Make a data set. The driving path image captured by the vehicle in automatic driving and intelligent monitoring is used as input, and the binary image of the edge of the manually marked image and the information of whether there is a tunnel are used as labels. The data is based on the input image, edge image and tunnel label. As a training sample, the data set consists of three parts: training set, verification set and test set.
[0045] S2. Load data in batches, load several samples each time, and crop the image size to the same size.
[0046] S3. Construct a loss function, calculate the cross-entropy loss of the 6 outputs of the network and the corresponding labels, and then add them together as the loss function. In this embodiment, the loss function is cross-entropy loss, and th...
PUM
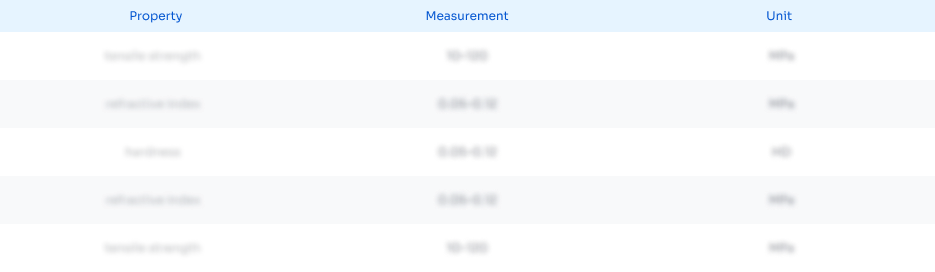
Abstract
Description
Claims
Application Information
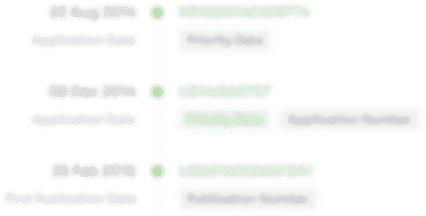
- R&D Engineer
- R&D Manager
- IP Professional
- Industry Leading Data Capabilities
- Powerful AI technology
- Patent DNA Extraction
Browse by: Latest US Patents, China's latest patents, Technical Efficacy Thesaurus, Application Domain, Technology Topic, Popular Technical Reports.
© 2024 PatSnap. All rights reserved.Legal|Privacy policy|Modern Slavery Act Transparency Statement|Sitemap|About US| Contact US: help@patsnap.com