Rolling bearing fault intelligent diagnosis method and system
An intelligent diagnosis, rolling bearing technology, applied in neural learning methods, testing of machine/structural components, testing of mechanical components, etc., can solve problems such as less data, difficult feature extraction, and difficult bearing fault diagnosis.
- Summary
- Abstract
- Description
- Claims
- Application Information
AI Technical Summary
Problems solved by technology
Method used
Image
Examples
Embodiment 1
[0062] Such as figure 1 with figure 2 As shown, it is a preferred implementation process and structure of a rolling bearing fault intelligent diagnosis method according to the present invention. The method is a rolling bearing fault intelligent diagnosis method based on transfer learning and deep learning, including the following steps:
[0063] Step S1, collection of test vibration signals: build a test bench, use the acceleration sensor to collect enough vibration signals of the bearing parts, and use the acceleration sensor to collect vibration signals under the actual working conditions of the equipment;
[0064] Step S2, preprocessing of the vibration signal: test the vibration signal and the corresponding state label set with D s and y s Indicates that the bearing vibration signal and its corresponding state label set under the actual working condition of the equipment are represented by D t and y t Indicates that the value range of each label is [0, K], indicating ...
Embodiment 2
[0123] A system for implementing the rolling bearing fault intelligent diagnosis method described in Embodiment 1, including a vibration signal acquisition module, a vibration signal preprocessing module, a source network construction and training module, a test signal and live signal similarity calculation module, and a target network construction and training module;
[0124] The vibration signal acquisition module is used to collect test signals and live signals of bearing vibration;
[0125] The vibration signal preprocessing module is used to perform 0-1 standardization processing on the test signal and live signal of bearing vibration, and divide the training set and the test set respectively;
[0126] The source network construction and training modules are used to construct the LSTM-softmax network, use the test signal to train the network, and obtain the source network suitable for the test;
[0127] The test signal and live signal similarity calculation module are u...
PUM
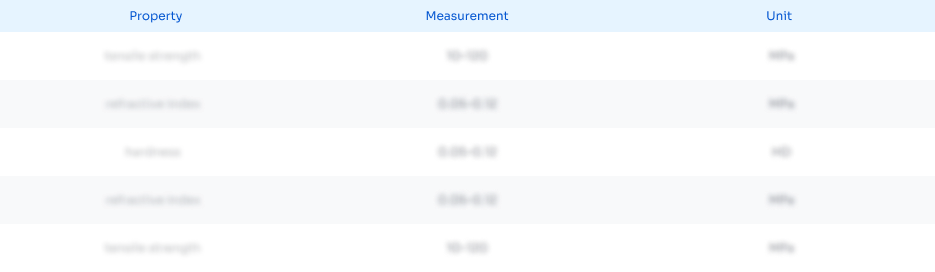
Abstract
Description
Claims
Application Information
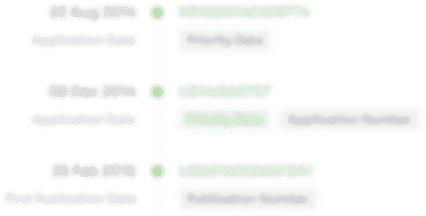
- R&D Engineer
- R&D Manager
- IP Professional
- Industry Leading Data Capabilities
- Powerful AI technology
- Patent DNA Extraction
Browse by: Latest US Patents, China's latest patents, Technical Efficacy Thesaurus, Application Domain, Technology Topic.
© 2024 PatSnap. All rights reserved.Legal|Privacy policy|Modern Slavery Act Transparency Statement|Sitemap