Fluid simulation data prediction model based on LSTM
A fluid simulation and data prediction technology, which is applied in electrical digital data processing, design optimization/simulation, biological neural network model, etc.
- Summary
- Abstract
- Description
- Claims
- Application Information
AI Technical Summary
Problems solved by technology
Method used
Image
Examples
Embodiment 1
[0053] An LSTM-based scroll compressor fluid simulation data prediction model is characterized in that: for the scroll compressor fluid, a combination of finite element simulation and LSTM data prediction is used to increase the fluid simulation speed, thereby improving scroll compression. The machine-fluid simulation process is characterized by:
[0054] (1) Perform a scroll compressor fluid simulation:
[0055] Firstly, a three-dimensional model of the fluid domain of the scroll compressor is established, and the dynamic mesh method based on the FLUENT software is used to perform a transient calculation of the three-dimensional flow field inside the scroll compressor. The internal transient flow field value at time, thus obtaining the distribution of internal pressure, temperature and velocity of the flow field under different working conditions;
[0056] (2) Establish three observation points at the Z=0.018m plane of the internal flow field of the scroll compressor, and ob...
Embodiment 2
[0062] This embodiment is basically the same as the first embodiment, and the special features are:
[0063] In this example, see figure 1 , use L1 regularization to reduce the complexity of the neural network model, and its loss function C is:
[0064]
[0065] In the above formula, the modified term sums the weights in the neural network, λ represents the regularization parameter, n is the number of samples contained in the training set, W represents the weight of the neuron, x is the input value of each neuron, and y is the Output value, α is the estimated value, L is the power of the estimated value, w is the weight of each neuron, and the partial derivative of C with respect to W can be obtained:
[0066]
[0067] In the above formula, sgn represents the symbolic function, C 1 is the original cost function, W represents the weight of the neuron; when W is greater than 0, the result is 1, and when W is less than 0, the result is -1; the update method of the weight ...
Embodiment 3
[0095] This embodiment is basically the same as the above-mentioned embodiment, and the special features are:
[0096] In this embodiment, as figure 1 As shown, an LSTM-based fluid simulation data prediction model includes the following steps:
[0097] Feed the time series data of the observation points into the LSTM neural network, first perform normalization processing, and map all the data to the interval 0-1;
[0098] Then continuously fine-tune the loss function and the regularized parameter values in the LSTM layer during model training;
[0099] Finally, the present invention adopts the absolute mean square error as an index to measure the error performance of the neural network.
[0100] In this embodiment, preprocessing is performed on the collected thermodynamic time series data of observation points, and the original data is normalized by using a discrete normalization processing method;
[0101]
[0102] where max is the maximum value of the sample data, an...
PUM
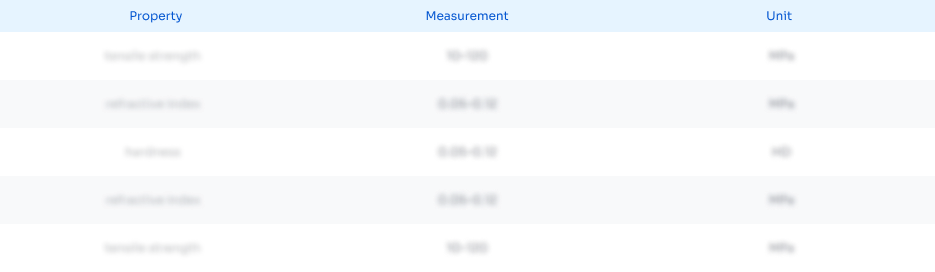
Abstract
Description
Claims
Application Information
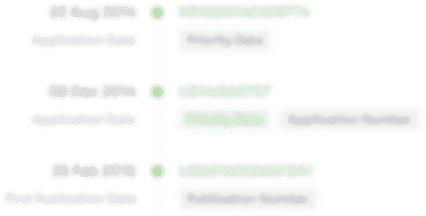
- R&D
- Intellectual Property
- Life Sciences
- Materials
- Tech Scout
- Unparalleled Data Quality
- Higher Quality Content
- 60% Fewer Hallucinations
Browse by: Latest US Patents, China's latest patents, Technical Efficacy Thesaurus, Application Domain, Technology Topic, Popular Technical Reports.
© 2025 PatSnap. All rights reserved.Legal|Privacy policy|Modern Slavery Act Transparency Statement|Sitemap|About US| Contact US: help@patsnap.com