Multi-vehicle target identification method based on improved YOLOv2 model
A target recognition, multi-vehicle technology, applied in the field of image detection and classification, can solve the problems of poor robustness, low detection rate, and unsatisfactory classification effect.
- Summary
- Abstract
- Description
- Claims
- Application Information
AI Technical Summary
Problems solved by technology
Method used
Image
Examples
Embodiment Construction
[0061] The present invention will be described in detail below in conjunction with the accompanying drawings and specific embodiments.
[0062] The present invention improves a multi-vehicle target recognition method of the YOLOv2 model, and the flow chart is as follows figure 1 As shown, the specific steps are as follows:
[0063] Step 1, collect the sample data under the actual traffic environment, and divide the sample data into the sample images of the training set and the test set with a ratio of 7:3;
[0064] Step 1 is as follows:
[0065] Step 1.1, shoot the vehicle information under the real-time road traffic environment, extract the captured video frame into image format and delete the pictures with poor image quality;
[0066] Step 1.2. Use the LabImage labeling tool to label the vehicles in the selected picture, frame the target area, and classify the vehicles in the target area and make labels. The labels are car, bus, van, truck, and each picture generates a .xm...
PUM
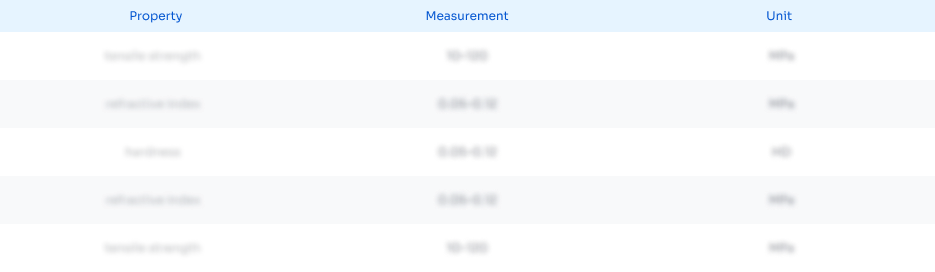
Abstract
Description
Claims
Application Information
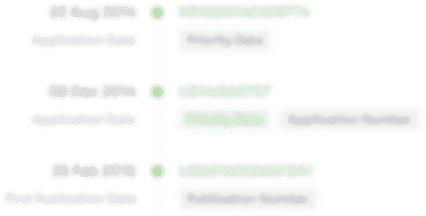
- R&D Engineer
- R&D Manager
- IP Professional
- Industry Leading Data Capabilities
- Powerful AI technology
- Patent DNA Extraction
Browse by: Latest US Patents, China's latest patents, Technical Efficacy Thesaurus, Application Domain, Technology Topic, Popular Technical Reports.
© 2024 PatSnap. All rights reserved.Legal|Privacy policy|Modern Slavery Act Transparency Statement|Sitemap|About US| Contact US: help@patsnap.com