Fruit picking robot target detection method based on deep learning in unstructured environment
A picking robot and target detection technology, which is applied in the field of target detection of fruit picking robots based on deep learning, can solve the problems of insufficient recognition accuracy, changing geometric feature growth cycle, and high computer hardware requirements, so as to reduce the difficulty of gradient disappearance and training. , the effect of reducing the amount of calculation and running time, good robustness and generality
- Summary
- Abstract
- Description
- Claims
- Application Information
AI Technical Summary
Problems solved by technology
Method used
Image
Examples
Embodiment Construction
[0043]The embodiments of the present invention are described below through specific specific examples, and those skilled in the art can easily understand other advantages and effects of the present invention from the contents disclosed in this specification. The present invention can also be implemented or applied through other different specific embodiments, and various details in this specification can also be modified or changed based on different viewpoints and applications without departing from the spirit of the present invention. It should be noted that the drawings provided in the following embodiments are only used to illustrate the basic idea of the present invention in a schematic manner, and the following embodiments and features in the embodiments can be combined with each other without conflict.
[0044] see Figure 1 to Figure 6 , the present invention optimizes a flow chart of a method for detecting the target of a tomato picking robot based on deep learning ...
PUM
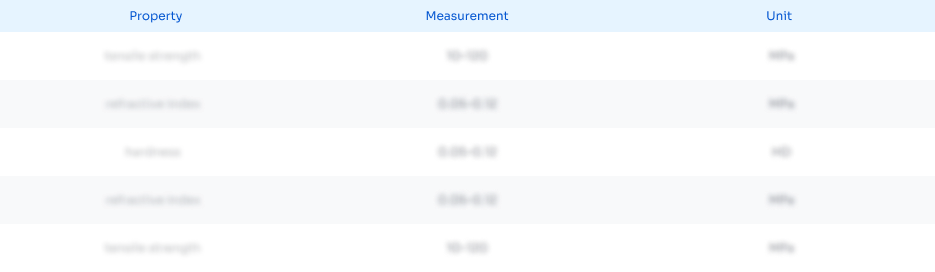
Abstract
Description
Claims
Application Information
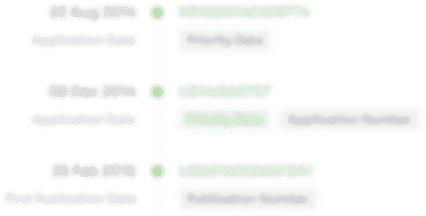
- R&D Engineer
- R&D Manager
- IP Professional
- Industry Leading Data Capabilities
- Powerful AI technology
- Patent DNA Extraction
Browse by: Latest US Patents, China's latest patents, Technical Efficacy Thesaurus, Application Domain, Technology Topic, Popular Technical Reports.
© 2024 PatSnap. All rights reserved.Legal|Privacy policy|Modern Slavery Act Transparency Statement|Sitemap|About US| Contact US: help@patsnap.com