Nasopharyngeal carcinoma radiotherapy target region automatic segmentation method based on deep neural network
A deep neural network, nasopharyngeal cancer radiotherapy technology, applied in neural learning methods, biological neural network models, neural architecture and other directions, can solve the problems of large individual differences between patients, affecting the accuracy of automatic delineation, etc., to improve work efficiency, speed up The effect of radiation therapy planning
- Summary
- Abstract
- Description
- Claims
- Application Information
AI Technical Summary
Problems solved by technology
Method used
Image
Examples
Embodiment
[0047] This embodiment provides a method for automatic segmentation of radiotherapy targets for nasopharyngeal carcinoma based on deep neural networks. The schematic diagram of the overall process is shown in figure 2 , this embodiment is different from the traditional manual feature-based segmentation method. The deep neural network will automatically learn how to extract task-related abstract features from the data. The extracted features have stronger expressive ability and higher translational accuracy. transsexual.
[0048] The segmentation method provided in this embodiment is roughly divided into three steps: data acquisition, model training and model verification.
[0049] In the specific application process, training a deep neural network often requires a large amount of labeled data, so the first step in the process is data acquisition, which specifically includes the following steps:
[0050] (1a) CT image acquisition: the tomographic image of the patient can be a...
PUM
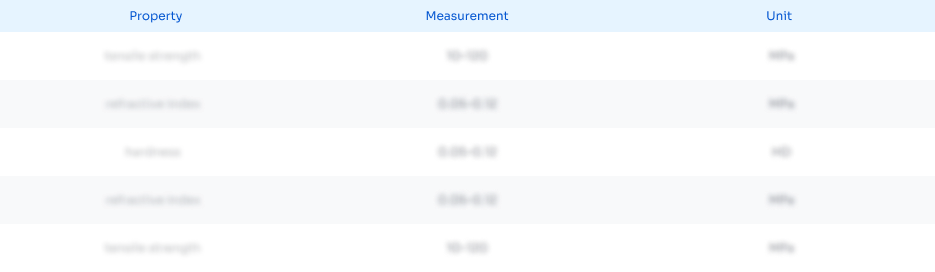
Abstract
Description
Claims
Application Information
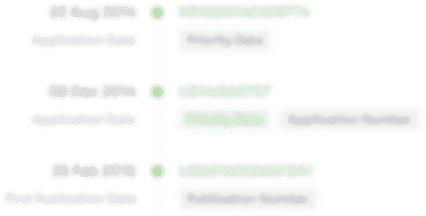
- R&D
- Intellectual Property
- Life Sciences
- Materials
- Tech Scout
- Unparalleled Data Quality
- Higher Quality Content
- 60% Fewer Hallucinations
Browse by: Latest US Patents, China's latest patents, Technical Efficacy Thesaurus, Application Domain, Technology Topic, Popular Technical Reports.
© 2025 PatSnap. All rights reserved.Legal|Privacy policy|Modern Slavery Act Transparency Statement|Sitemap|About US| Contact US: help@patsnap.com