Underwater image enhancement method based on multi-residual joint learning
An underwater image and residual technology, applied in the field of deep learning, can solve problems such as not fully adapting to the underwater environment, and achieve the effect of improving serious color cast, reducing color cast, and improving quality
- Summary
- Abstract
- Description
- Claims
- Application Information
AI Technical Summary
Problems solved by technology
Method used
Image
Examples
Embodiment 1
[0033] In this embodiment, the underwater image enhancement method based on multi-residual joint learning includes the following steps:
[0034] S100, randomly cutting pictures of different resolutions in the underwater image data set including the degraded image and the corresponding reference image into images of the same resolution, and establishing a training set for the underwater image enhancement model;
[0035] S200, processing the cropped degraded images in the training set using multiple preprocessing methods, each preprocessing method correspondingly obtains a preprocessed image;
[0036] S300, using the reference image as the label of the degraded image, inputting the original image of the degraded image and the preprocessed degraded image into a multi-branch convolutional neural network of multi-residual joint learning for training to obtain an image enhancement model;
[0037] S400. Input the image to be enhanced into the image enhancement model to obtain a proce...
Embodiment 2
[0073] In this embodiment, except that the structure of the convolutional neural network is different from that of Embodiment 1, the rest are the same as Embodiment 1, and will not be repeated here.
[0074] In step S300 of this embodiment, firstly, a multi-residual joint learning generative adversarial network model is designed to enhance the underwater image and eliminate the blue-greenish phenomenon of the underwater image. The generator of the multi-residual joint learning generative adversarial network includes a convolutional network unit, a residual network unit, and a channel attention module.
[0075] The first branch: the input image is a cropped original image with a size of 256×256. Starting from the convolution stage, there is a first convolution unit and a second convolution unit for downsampling, which are used to learn the low-frequency information of the image; the first convolution unit and the second convolution unit are followed by several The residual gro...
PUM
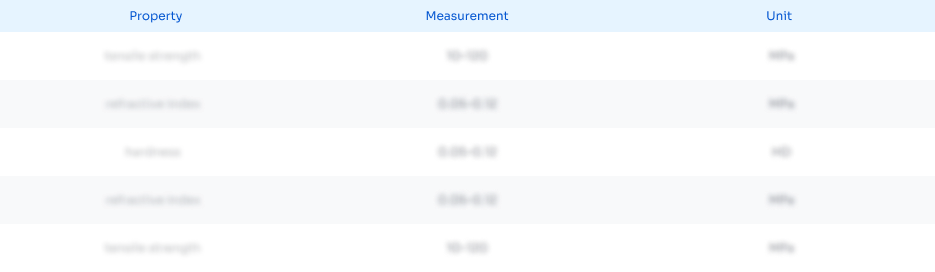
Abstract
Description
Claims
Application Information
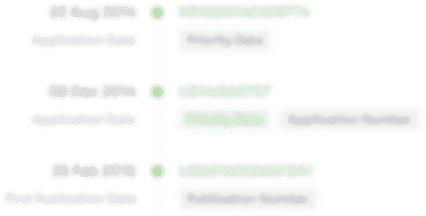
- R&D
- Intellectual Property
- Life Sciences
- Materials
- Tech Scout
- Unparalleled Data Quality
- Higher Quality Content
- 60% Fewer Hallucinations
Browse by: Latest US Patents, China's latest patents, Technical Efficacy Thesaurus, Application Domain, Technology Topic, Popular Technical Reports.
© 2025 PatSnap. All rights reserved.Legal|Privacy policy|Modern Slavery Act Transparency Statement|Sitemap|About US| Contact US: help@patsnap.com