Retinal neuron coding method based on convolutional neural network
A technology of convolutional neural network and coding method, applied in the field of coding of single ganglion cells in the retina, can solve problems such as unclear CNN learning and poor natural scene coding ability
- Summary
- Abstract
- Description
- Claims
- Application Information
AI Technical Summary
Problems solved by technology
Method used
Image
Examples
Embodiment 1
[0060] In the LNLN model, the calculation components such as temporal filters, spatial receptive fields, nonlinear functions, and connection weights of each layer are known. After a white noise stimulus is given, the corresponding simulated impulse response is obtained. Using such a simulation It is found that the CNN model trained by the data can not only predict the response well, but also find that the first layer of convolution kernel just corresponds to the spatiotemporal receptive field of the first layer of subunits in the simulation model.
[0061] The CNN model trained with real physiological data can also restore the receptive field of bipolar cells in real data. Specifically, the CNN model can identify the spatiotemporal receptive field of the subunit in the ganglion cell model through the input stimulus image and the output response pulse.
Embodiment 2
[0063] According to the CNN model of the specified ganglion cells (training set) trained according to specific stimuli (white noise or natural images), when a new visual stimulus with the same distribution (test set, which belongs to the same type as the data in the training set) is input, CNN models can predict neural responses very well. For example, a CNN model trained using the white noise data of ganglion cell A can predict the response of ganglion cell A to new white noise stimuli; similarly, a CNN model trained using natural image data of ganglion cell B can predict Predict the response of this ganglion cell B to novel natural image stimuli.
Embodiment 3
[0065] Given some ganglion cell data and a trained CNN model, you can consider studying the migration learning ability or generalization ability of the CNN model. That is, the CNN model trained with the data of one ganglion cell predicts the response of another cell. For example, the CNN model trained by using the response of neuron C to white noise stimulation can not only accurately predict the response of neuron C to new white noise stimulation, but also predict the response of neuron D to new white noise stimulation.
PUM
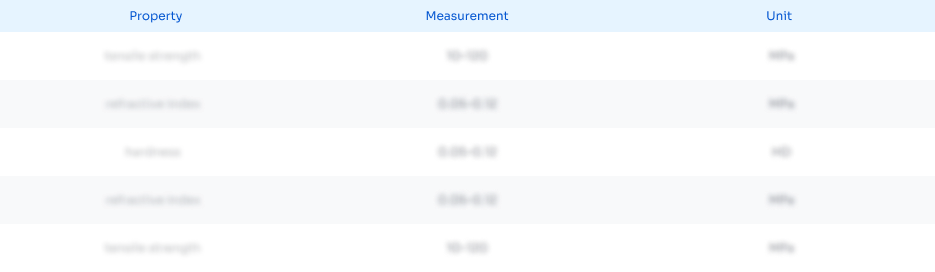
Abstract
Description
Claims
Application Information
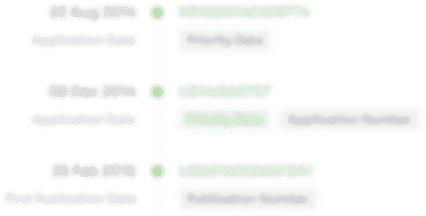
- Generate Ideas
- Intellectual Property
- Life Sciences
- Materials
- Tech Scout
- Unparalleled Data Quality
- Higher Quality Content
- 60% Fewer Hallucinations
Browse by: Latest US Patents, China's latest patents, Technical Efficacy Thesaurus, Application Domain, Technology Topic, Popular Technical Reports.
© 2025 PatSnap. All rights reserved.Legal|Privacy policy|Modern Slavery Act Transparency Statement|Sitemap|About US| Contact US: help@patsnap.com