Continuous intersection signal cooperative control method based on deep reinforcement learning
A technology of reinforcement learning and collaborative control, applied in the traffic control system of road vehicles, traffic control system, control of traffic signals, etc., can solve the problem of low coordination efficiency of intersection signals, difficult to guarantee the practicability and operability of algorithms, large computing issues such as quantity
- Summary
- Abstract
- Description
- Claims
- Application Information
AI Technical Summary
Problems solved by technology
Method used
Image
Examples
Embodiment Construction
[0074] Step 1. The framework of the signal control model for the upper and lower layers of continuous intersections
[0075] Establishment of continuous intersections The upper and lower traffic signal intersection scenes are established. The continuous intersections include detectors for detecting vehicle information, and sensors for obtaining information are also installed on the vehicles. Real-time acquisition of traffic signal timing data, vehicle driving status, and actual road conditions through in-vehicle network technology and various sensors, and then using deep reinforcement learning to predict signal timing that meets the current traffic status through neural networks.
[0076] The present invention divides the control of continuous intersection signal lights into upper and lower levels of control. The lower level Agent is the traffic signal controller of each intersection, and each controller has its own learning strategy; the upper level Agent is mainly used to adj...
PUM
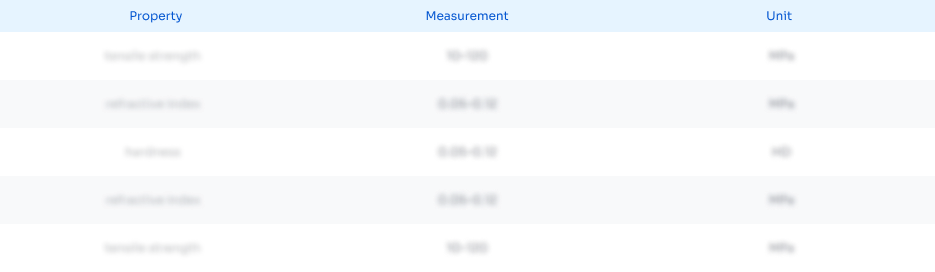
Abstract
Description
Claims
Application Information
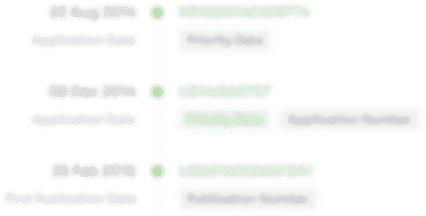
- R&D
- Intellectual Property
- Life Sciences
- Materials
- Tech Scout
- Unparalleled Data Quality
- Higher Quality Content
- 60% Fewer Hallucinations
Browse by: Latest US Patents, China's latest patents, Technical Efficacy Thesaurus, Application Domain, Technology Topic, Popular Technical Reports.
© 2025 PatSnap. All rights reserved.Legal|Privacy policy|Modern Slavery Act Transparency Statement|Sitemap|About US| Contact US: help@patsnap.com