Syntactic relationship enhanced machine reading understanding multi-hop reasoning model and method
A technology of reading comprehension and reasoning methods, which is applied in the field of machine reading comprehension multi-hop reasoning models, which can solve problems such as poor performance of answering methods and inaccurate answer basis, and achieve the effect of improving interpretability and improving answering methods
- Summary
- Abstract
- Description
- Claims
- Application Information
AI Technical Summary
Problems solved by technology
Method used
Image
Examples
Embodiment 1
[0073] figure 1 It is a model frame diagram of the present invention, which mainly includes a text coding module, an associated element relationship diagram building module, a question answering module, and an answer prediction module, wherein the question answering module includes an answer generation module and a multi-hop reasoning module, and the specific contents of each module are as follows:
[0074] A text encoding module encodes the questions and articles to obtain semantic representations of the questions and articles;
[0075] Associating element relationship diagram construction module, identifying the key elements in each sentence of the article and the dependent syntactic relationship between them, defining element association rules, and using the association rules to construct the association element relationship diagram;
[0076] The multi-hop reasoning module performs multi-hop reasoning based on the relational element relationship graph and the graph attentio...
Embodiment 2
[0080] figure 2 An example from the reading comprehension dataset for the 2020 China "Law Research Cup" Judicial Artificial Intelligence Challenge (CAIL2020). Such as figure 2 As mentioned above, the article is a real case in Chinese judgment documents, the question is "Is there a time limit in the contract?", the answer is "yes", and the answer is based on the sentence numbers "4, 6" in the article.
[0081] 1. First, use the text encoding module to encode the questions and articles, and obtain the semantic vectorized representations of the questions and articles. The present invention uses the RoBERTa model as an encoder to map the article and each word or phrase of the article to a high-dimensional vector space to obtain the semantic representation of each word or word. The calculation formula is as follows:
PUM
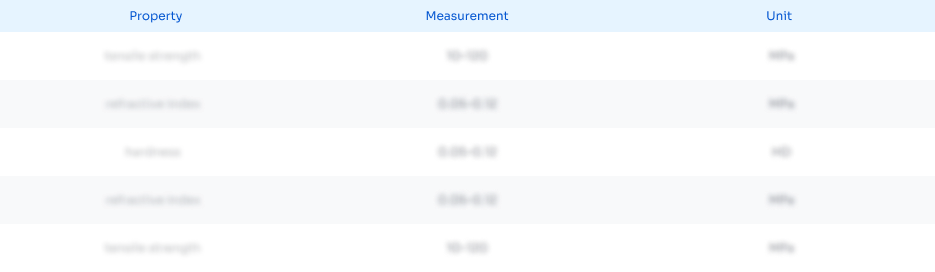
Abstract
Description
Claims
Application Information
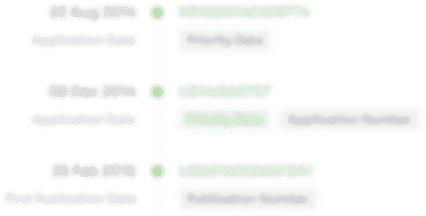
- R&D Engineer
- R&D Manager
- IP Professional
- Industry Leading Data Capabilities
- Powerful AI technology
- Patent DNA Extraction
Browse by: Latest US Patents, China's latest patents, Technical Efficacy Thesaurus, Application Domain, Technology Topic, Popular Technical Reports.
© 2024 PatSnap. All rights reserved.Legal|Privacy policy|Modern Slavery Act Transparency Statement|Sitemap|About US| Contact US: help@patsnap.com